Teach me STATISTICS in half an hour! Seriously.
zedstatistics・2 minutes read
Justin Zeltzer explains statistics in under half an hour, covering categorical and numerical data, sample statistics, hypothesis testing, and the importance of p-values in drawing statistical conclusions without using the words "prove" or "accept." Parameters like theta, mu, and sigma are estimated from samples, and p-values help determine the probability of extreme results to support rejecting the null hypothesis.
Insights
- Statistics can categorize data into categorical (nominal or ordinal) and numerical (discrete or continuous), showcasing different ways to analyze and interpret information.
- Hypothesis testing in statistics involves setting a null hypothesis, determining a rejection region based on significance levels, and using p-values to assess the likelihood of obtaining extreme results, emphasizing the importance of methodical and precise research practices to avoid misinterpretation and false conclusions.
Get key ideas from YouTube videos. It’s free
Recent questions
What are the two main categories of data in statistics?
Categorical and numerical data.
How can proportions be derived from nominal data in statistics?
Proportions are numerical data derived from nominal data.
How are sampling distributions used in statistical analysis?
Sampling distributions show the probability distribution of averages from a sample.
What is the purpose of hypothesis testing in statistics?
Hypothesis testing involves assessing sample evidence against the null hypothesis.
How are p-values used in hypothesis testing?
P-values determine the probability of obtaining results as extreme as the sample.
Related videos
Tech Classes
Complete STATISTICS for Data Science | Data Analysis | Full Crash Course
VVC Schellhous
Math 120: Statistics --- Chapter 1: Definitions and Data
Mr. Sinn
AP Psychology Unit 1 Review [Everything You NEED to Know]
Khan Academy
Statistical questions | Data and statistics | 6th grade | Khan Academy
Bozeman Science
Chi-squared Test
Summary
00:00
"Explaining Statistics in Under 30 Minutes"
- Justin Zeltzer from zstatistics.com responds to a challenge to explain statistics in under half an hour.
- The introduction to statistics is done without math and in under half an hour.
- Data in statistics can be categorized into categorical and numerical data.
- Categorical data can be nominal or ordinal, while numerical data can be discrete or continuous.
- Examples include Steph Curry's team as categorical data and his missed free throws as discrete numerical data.
- Steph Curry's height is an example of continuous numerical data.
- Proportions, like Steph's three-point percentage, are numerical data derived from nominal data.
- The distribution of NBA players' heights can be represented by a probability density function.
- Sampling distributions show the probability distribution of averages from a sample.
- Statistics aims to estimate unknown values like Steph Curry's true three-point percentage using sample statistics.
16:09
Quantifying Uncertainty in Statistical Inferences
- Statisticians create 95% confidence intervals to quantify uncertainty in sample estimates.
- Meyers Leonard, with a 0.6 three-point percentage, may seem a better shooter than Steph Curry, but his confidence interval is wider due to a smaller sample size.
- Parameters like theta (long-term three-point percentage) are estimated from samples, denoted by Greek letters like mu (mean) and sigma (standard deviation).
- Sample statistics, like x-bar for mean height, estimate parameters like theta.
- Hypothesis testing involves setting a null hypothesis (H naught) and an alternate hypothesis to assess sample evidence against the null.
- A rejection region is determined based on the level of significance (often 5%) to reject the null hypothesis if the sample statistic falls within it.
- Hypothesis tests do not prove but infer conclusions; never use the words "prove" or "accept" in statistical conclusions.
- P-values are used to determine the probability of obtaining results as extreme as the sample, given the null hypothesis.
- Not rejecting the null hypothesis does not mean accepting it; it signifies a lack of evidence to support the alternate hypothesis.
- The distinction between innocence and not guilty in legal systems mirrors the statistical concept of not rejecting the null hypothesis.
31:58
"P-value: Rejecting Null Hypothesis in Testing"
- The p-value measures how extreme a sample is in hypothesis testing, determining if the null hypothesis should be rejected.
- In an example with Meyers Leonard's three-point percentage, a test statistic of 9 out of 15 was not extreme enough to reject the null hypothesis.
- The p-value calculates the region where the test statistic lies, indicating how much of the distribution is at or above the test statistic.
- A small p-value suggests a more extreme sample, increasing the likelihood of rejecting the null hypothesis.
- If the p-value drops below 0.05, the test statistic must be in the rejection region, leading to the null hypothesis rejection.
- P-hacking involves misusing p-values in research, potentially invalidating scientific findings by selecting significant results from multiple tests.
- Good research theorizes a specific effect, collects data to test that effect, and concludes based on a low p-value, while bad research tests multiple effects and selects significant results post hoc, a practice prone to false positives.


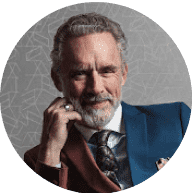
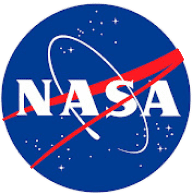
