Math 120: Statistics --- Chapter 1: Definitions and Data
VVC Schellhous・5 minutes read
Statistics involves organizing, analyzing, and interpreting data to draw conclusions, emphasizing the importance of good data for quality results in statistical studies. Different types of data, such as quantitative and qualitative, along with levels of measurement, play a crucial role in statistical analysis and interpretation.
Insights
- Statistics involves two main branches: descriptive statistics, which focuses on organizing and summarizing data using tools like charts, and inferential statistics, which goes beyond description to draw conclusions through formal methods like hypothesis tests.
- Sampling methods are crucial in statistics, with the gold standard being a simple random sample, although difficult to obtain. Other methods like systematic, convenience, stratified, and cluster sampling offer structured approaches to gathering representative data from populations.
Get key ideas from YouTube videos. It’s free
Recent questions
What is the importance of vocabulary in statistics?
Vocabulary is crucial in statistics as it aids in understanding concepts. Writing down new words and their meanings helps in grasping statistical principles and applying them effectively.
What are the phases of a statistical study?
Statistics involves preparing, analyzing, and concluding in a study. A project in the class typically follows these phases, emphasizing the importance of thorough planning, data analysis, and drawing meaningful conclusions.
How are populations and samples used in statistics?
Populations represent all data, while samples are smaller subsets used for study and inference. Understanding the distinction between populations and samples is fundamental in statistical analysis and drawing accurate conclusions.
What are the differences between descriptive and inferential statistics?
Descriptive statistics organize and summarize data using mathematical tools like charts and formulas, focusing on presenting data effectively. In contrast, inferential statistics go beyond describing data to drawing conclusions using formal methods like hypothesis tests, emphasizing the importance of making inferences based on data.
Why is good data vital in statistical studies?
Good data is essential for statistical studies as it forms the foundation for accurate analysis and interpretation. The first chapter of a statistics course often emphasizes the importance of designing studies to obtain quality data, highlighting the significance of reliable data in drawing meaningful conclusions.
Related videos
Summary
00:00
Essential Statistics: Data, Analysis, and Interpretation
- Data is a significant topic in statistics, focusing on measuring data, types of data, and its applications.
- Taking notes during lectures is recommended to aid in homework and test preparation.
- Vocabulary is crucial in understanding statistics, with emphasis on writing down new words and their meanings.
- Statistics involves preparing, analyzing, and concluding in a study, with a project in the class following these phases.
- Statistical thinking is essential, focusing on critical thinking and interpreting results rather than just computations.
- Descriptive statistics, covered in the first half of the class, organizes and summarizes data using mathematical tools like charts and formulas.
- Inferential statistics, discussed in the second half, goes beyond describing data to drawing conclusions using formal methods like hypothesis tests.
- Good data is vital for statistical studies, with the first chapter emphasizing the importance of designing studies for quality data.
- Statistics is the science of planning, collecting, analyzing, and interpreting data to draw conclusions, used across various scientific fields.
- Populations and samples are fundamental in statistics, with populations representing all data and samples being smaller subsets used for study and inference.
17:36
Understanding Data Types for Statistical Analysis
- Mail-in polls are discussed, highlighting the issue of self-selection bias due to people deciding whether to reply, leading to a non-representative sample of strong opinions.
- Telephone call-in polls, like American Idol voting, are mentioned, emphasizing that those willing to participate likely have strong opinions, skewing results.
- The distinction between population and sample in statistics is explained, with most concepts having two names based on whether they pertain to a population or a sample.
- Statistics are used to draw conclusions about populations from sample data, exemplified by detecting failures in a sample of detectors and extrapolating to the entire population.
- Parameters and statistics are defined as numerical measurements describing characteristics of populations and samples, respectively, with examples like average height or GPA.
- Variables are introduced as the measured characteristics, such as height or GPA, with data representing the actual values collected for these variables.
- A word problem involving high school students is presented, distinguishing between parameters (population size) and statistics (sample size and percentage of students texting while driving).
- The difference between quantitative (numerical) and categorical (qualitative) data is explained, with quantitative data consisting of numbers representing counts or measurements.
- Examples of variables like age (quantitative), gender (qualitative), car mileage (quantitative), car color (qualitative), and shirt numbers of football players (quantitative) are used to illustrate the distinction.
- The importance of understanding the types of data collected, whether quantitative or qualitative, is emphasized for statistical analysis and interpretation.
35:00
Understanding Quantitative Data and Measurement Levels
- Jersey numbers, social security numbers, and student ID numbers are examples of numbers that do not measure anything about the players or individuals; they are merely labels.
- Quantitative data is essential for mathematical analysis, allowing for various calculations and comparisons.
- Quantitative data can be further categorized into discrete and continuous data.
- Discrete data consists of a finite number of countable values, where there is always a next value in line.
- Continuous data includes an infinite number of possible values, where decimal numbers make sense as answers.
- Examples of discrete data include a person's age, which is always a whole number, and the number of siblings one has.
- Continuous data examples include the exact height of a person and the distance a person commutes to work, where decimal values are applicable.
- Levels of measurement in data include nominal, ordinal, interval, and ratio, each representing increasing levels of precision.
- Nominal data consists of names or labels without a natural order, like survey responses or favorite colors.
- Ordinal data has a natural order but does not allow for meaningful subtraction, such as course grades or drink sizes.
52:49
Importance of Data Quality in Statistical Studies
- Data quality is crucial, as conclusions are only as good as the data collected.
- Data is typically obtained from observational studies and experiments.
- An experiment involves applying a treatment and observing the outcome.
- Experimental units are individuals in an experiment, often called subjects.
- Observational studies involve observing and measuring without modifying individuals.
- An example illustrates the differences between observational studies and experiments.
- Experiments are better at determining cause and effect relationships than observational studies.
- Sampling methods are essential for statistical studies, as studying the entire population is impractical.
- The gold standard in statistics is a simple random sample, although difficult to obtain.
- Commonly used sampling methods include systematic, convenience, stratified, and cluster sampling.
01:09:28
Sampling Methods in Research Studies
- Systematic sampling involves selecting every 10th house on a street, creating a structured sampling method.
- Multi-stage sampling combines various sampling techniques, reflecting real-life scenarios.
- Sampling with replacement allows for individuals to be selected more than once, increasing the likelihood of repeated selections.
- Sampling without replacement ensures that individuals are not selected more than once, enhancing the diversity of the sample.
- Sampling with replacement is suitable for large populations, with the general rule being that the population should be at least 20 times larger than the sample size for this method to be acceptable.


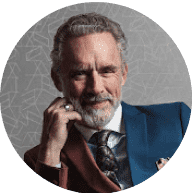
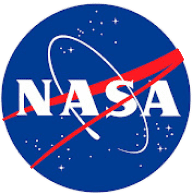
