Complete STATISTICS for Data Science | Data Analysis | Full Crash Course
Tech Classes・2 minutes read
The video covers comprehensive statistics topics, including probable inferential statistics and types of data, aiding in interview preparation. It explains descriptive and inferential statistics, different data types, variable types, measures of central tendency, probability, hypothesis testing, estimation, and Anova tests.
Insights
- The video comprehensively covers Statistics for data analysis and data science topics, aiding in interview preparation and serving as last-minute notes.
- Descriptive statistics focus on understanding data features through collection, analysis, and interpretation, while inferential statistics involve drawing conclusions from data samples to represent entire populations.
- Variables types like nominal, ordinal, numerical, categorical, interval, and ratio are explained, with examples provided for each type.
- Measures of central tendency, such as mean, median, and mode, along with measures of dispersion like range, quartiles, and percentiles, provide insights into data spread and variability.
- Hypothesis testing includes comparing sample data to a hypothesis about a population parameter, involving one-tail and two-tail tests with z-tests, t-tests, chi-square, and ANOVA used based on data and hypotheses.
Get key ideas from YouTube videos. It’s free
Recent questions
What is the difference between descriptive and inferential statistics?
Descriptive statistics focus on summarizing and interpreting data, while inferential statistics involve drawing conclusions and making predictions about populations based on sample data. Descriptive statistics help in understanding the characteristics of data, such as mean, median, and mode, while inferential statistics use sample data to make inferences about the entire population.
How are variables classified in statistics?
Variables in statistics are classified into different types, including nominal, ordinal, numerical, and categorical variables. Nominal variables represent categories without any order, ordinal variables have a specific order or ranking, numerical variables consist of numerical values, and categorical variables represent categories that can be counted or grouped.
What is the importance of sampling techniques in statistics?
Sampling techniques are crucial in statistics as they help in selecting representative samples from populations for analysis. Different sampling methods like random, stratified, systematic, and clustered sampling ensure that the sample accurately reflects the population, influencing the validity and reliability of statistical conclusions.
How are measures of central tendency calculated in statistics?
Measures of central tendency, such as mean, median, and mode, are calculated to understand the central values of a dataset. The mean is the average value obtained by summing all values and dividing by the count, the median is the middle value when data is arranged in order, and the mode is the most frequently occurring value in the dataset.
What is the significance of hypothesis testing in statistics?
Hypothesis testing is essential in statistics to make decisions based on sample data and hypotheses about population parameters. It involves comparing sample data to a hypothesis, determining the likelihood of observing the results if the null hypothesis is true, and making decisions about accepting or rejecting the null hypothesis based on statistical significance.
Related videos
VVC Schellhous
Math 120: Statistics --- Chapter 1: Definitions and Data
CA Foundation Grooming Education
Statistical Description of Data | CA Foundation Jan/May 25 | Quantitative Aptitude Chapter 13 | MSLR
The Organic Chemistry Tutor
Introduction to Statistics
Shady Attia
Statistical Analysis for Experimental Research
zedstatistics
Teach me STATISTICS in half an hour! Seriously.
Summary
00:00
"Statistics for Data Analysis and Science"
- The video covers Statistics for data analysis and data science topics comprehensively.
- Detailed notes are available in the video description for reference.
- The topics covered include Probable Inferential Statistics with real-life examples.
- The video aids in interview preparation and serves as last-minute notes.
- Introduction to Statistics is the first topic covered, followed by Descriptive Statistics.
- Types of statistics, including descriptive and inferential, are explained.
- Descriptive statistics focus on understanding data features through collection, analysis, and interpretation.
- Inferential statistics involve drawing conclusions from data samples to represent entire populations.
- Different types of data, such as structured and unstructured, cross-sectional, and time series, are discussed.
- The video delves into variable types like nominal, ordinal, numerical, categorical, interval, and ratio variables.
18:14
Types and Variables in Data Analysis
- Education levels can be 10th or 12th, with graduation or post-graduation following in order.
- Ordinal variables are exemplified by customer ratings, ranging from one to five.
- Numerical variables contain integer or float values, either discrete or continuous.
- Examples of numerical variables include income, age, and price.
- Data types include nominal, ordinal, interval, and ratio, with categories like types of cars or products.
- Interval data provides meaningful information without a true zero point, like temperature or IQ.
- Ratio data includes a true zero point, such as height or weight.
- Population refers to the entire group, while a sample is a subset representing the population.
- Sampling techniques include random, stratified, systematic, and clustered methods.
- Factors influencing sampling technique choice include population nature, research objectives, and available resources.
37:44
Importance of documenting and analyzing statistical data.
- Documenting the process is crucial for sharing and reporting on completed work, including hypothesis, solutions, and conclusions.
- Statistics play a key role in projects, aiding in comparisons and presentations.
- Descriptive statistics focus on measures of central tendency, such as mean, median, and mode.
- The mean is calculated by summing all values and dividing by the count of values, but it is sensitive to outliers.
- Outliers can heavily skew mean values, impacting the accuracy of results.
- The median, the middle value in a dataset, is less influenced by outliers and is more effective when extreme values are present.
- In cases of even numbers of values, the median is the average of the two middle values.
- The mode, the most frequently occurring value in a dataset, is useful for categorical variables.
- Measures of dispersion, like range, quartiles, and percentiles, provide information on the spread and variation of data.
- Quartiles divide data into four equal parts, while percentiles divide data into 100 equal parts, offering insights into data distribution and variability.
55:46
Calculating Percentiles and Analyzing Data Distribution
- Percentile calculation involves turning p into 100 using a formula.
- Quartiles calculation involves dividing by 100n+1 to find the 25th percentile.
- The formula for the 50th percentile is n+1/2.
- Percentile values can be calculated based on the number of observations.
- Interquartile range focuses on the middle 50% of data.
- Extreme values have minimal impact on interquartile range.
- Variance is calculated by mean square deviation from the mean.
- Variance formula involves summing the squared deviations from the mean.
- Standard deviation is the square root of the variance.
- Frequency and relative frequency help analyze data distribution and occurrence.
01:12:29
Understanding Probability Distributions and Data Relationships
- Distributions in probability are visually represented by histograms, including Cymatic, Right Skewed, and Left Skewed histograms.
- Normal distribution in data signifies a symmetrical histogram with equal distribution on both sides of the center.
- Mean, median, and mode are central values in a distribution, with normal distribution having these values close to each other.
- Right Skewed histograms show outliers on the right side, with the mean being greater than the median.
- Left Skewed histograms display outliers on the left side, with the median being greater than the mean.
- Histograms can be categorized based on the number of modes, including Uni-modal, Bi-modal, and Multi-modal histograms.
- Box plots, also known as whisker plots, represent the spread of data with the interquartile range and median.
- Scatter plots visually depict the relationship between two continuous variables, showing positive, negative, or no correlation.
- Outliers in data can significantly impact results, with methods like z-scores and interquartile range used for outlier identification and removal.
- Covariates describe how two variables change together, indicating the direction and strength of their relationship, whether positive or negative.
01:31:29
Understanding Co-relation and Probability in Analysis
- Co-relation describes the strength between two variables, showing the direction of the relationship as positive, negative, or neutral.
- Positive co-relation indicates that as one variable increases, the other also increases, while negative co-relation means one variable increases as the other decreases.
- A value closer to 1 signifies a strong positive co-relation, while a value near -1 indicates a strong negative co-relation.
- If the co-relation value is between 0 and 0.5, it suggests a positive relationship, while -0.9 signifies a strong negative co-relation.
- The formula for co-relation involves the standard deviation of x and y, as well as the variance equation.
- Causation refers to the direct relationship between cause and effect, distinct from co-relation which only shows the relationship between variables.
- Outliers in data can impact the co-relation coefficient, affecting the relationship between variables.
- Co-relation is a measure of the strength of a linear relationship, not a judgment of the relationship itself.
- An example of co-relation and variance in the stock market analysis demonstrates how to assess the relationship between two variables.
- Probability is a measure of the likelihood of an event occurring, ranging from 0 (impossible) to 1 (certain), with values in between indicating varying degrees of likelihood.
01:51:48
Dependent and Independent Events in Probability
- If a red card is drawn from a deck of 52 cards, leaving 51 cards.
- The outcome of drawing a red card is 51, with no effect on black cards.
- Event B is dependent on Event A, affecting probability calculations.
- Dependent events in probability involve calculating probabilities based on previous events.
- Independent events do not affect each other's outcomes.
- Drawing a red card and a face card involves calculating joint event probabilities.
- The probability of drawing a king and a queen is 4/52.
- Conditional probability is based on previous events affecting future probabilities.
- The Bayes Theorem updates probabilities based on new evidence.
- The Bayes Theorem is crucial in machine learning applications like medical diagnoses and spam classification.
02:09:23
Probability Distributions in Data Analysis
- Visual representation of probabilities using bar charts for discrete random variables
- Probability mass function for tossing four coins: 1/1, 2/6, 4/1, 6/6, 16/4, 1/1
- Example of a probability density function for a call center's wait time
- Calculation of probability density for customers waiting less than 5 minutes
- Introduction to Bernoulli distribution with binary outcomes
- Probability mass function for Bernoulli distribution: p if x=1, 1-p if x=0
- Explanation of binomial distribution with multiple Bernoulli trials
- Calculation of probability for a specific number of purchases in an e-commerce scenario
- Application of uniform distribution in generating random values
- Introduction to normal distribution with a bell-shaped curve and its probability density function formula
02:24:51
Understanding Normal Distribution and Standardization in Statistics
- A blue histogram represents data in a histogram format.
- The y-axis of a histogram represents probability density.
- A black curve signifies the Probability Density Function.
- A normal distribution is indicated by a completely normal distribution.
- Standard deviation is calculated by adding or subtracting sigma values from the mean.
- Standard normal distribution is achieved by converting each value into z scores.
- Standardization converts normal distribution into standard normal distribution.
- Normalization rescales a dataset to fall between zero and one.
- Standardization uses mean and standard deviation for scaling.
- The empirical rule applies to normal distribution, with data percentages within one, two, and three standard deviations from the mean.
02:40:24
Drawing Inferences and Making Predictions with Statistics
- Inferential statistics involves drawing conclusions or inferences about a population based on a sample.
- Descriptive statistics helps understand the nature of data, identifying patterns and trends.
- Predictions about a population are made using sample data.
- Estimation involves making estimates about population parameters based on sample statistics.
- Point estimation provides a single best guess for an unknown population parameter.
- Consistency in point estimation ensures that estimates are close to the actual parameter.
- Bias should be avoided in estimation by using diverse samples representing the population.
- Interval estimation provides a range of values instead of a single estimate, increasing reliability.
- Confidence intervals indicate the probability that the true population parameter lies within a computed interval.
- Calculating the confidence interval involves determining the point estimate, margin of error, and critical values.
02:57:06
"Statistics Essentials: Confidence, Margin, Hypothesis, Errors"
- Confidence interval is determined by alpha value, with 0.05 commonly used.
- Margin of error is calculated using critical values from z and t tables.
- Sample size should be greater than 30 for z distribution and less than or equal to 30 for t distribution.
- Z score is calculated as (x - u) / sigma for standard normal distribution.
- Sample standard deviation (s) is used when population standard deviation is unknown.
- Degrees of freedom in t distribution are represented by n - 1.
- Hypothesis testing involves comparing sample data to a hypothesis about a population parameter.
- Null hypothesis is the default position, while alternative hypothesis is the opposite.
- Decision rule: if p value is less than alpha, null hypothesis is rejected.
- Type one error occurs when null hypothesis is rejected incorrectly, while type two error occurs when null hypothesis is accepted incorrectly.
03:14:10
Understanding Errors and Tests in Hypothesis Testing
- Type I and Type II errors occur when accepting or rejecting a null hypothesis, leading to incorrect decisions.
- Type I error happens when a null hypothesis is true, but it is rejected, while Type II error occurs when a false null hypothesis is accepted.
- An example of Type I error is diagnosing a healthy patient with a disease, and Type II error is diagnosing a patient with a disease as healthy.
- Hypothesis testing involves one-tail and two-tail tests, with one-tail tests used for directional effects.
- One-tail tests have critical regions on either the right or left side of a distribution, depending on the hypothesis.
- Two-tail tests have critical regions on both sides of the distribution, dividing the significance level.
- Tests in hypothesis testing include z-tests, t-tests, chi-square, and ANOVA, each used based on data and hypotheses.
- Z-tests are used when the population standard deviation is known and for large sample sizes.
- T-tests are used when the population standard deviation is unknown and for small sample sizes.
- ANOVA is used to compare means of more than two groups, with F-value calculated to determine significance.
03:31:06
Comparing samples with Anova: A guide
- One-way Anova involves comparing samples with one factor variable and one response variable.
- The response variable in one-way Anova depends on the factor variable.
- An example of one-way Anova is comparing three classes with different teachers teaching the same subject.
- Hypotheses in one-way Anova include the null hypothesis of equal means and alternative hypotheses of inequality.
- Two-way Anova differs from one-way Anova by having two factor variables influencing the response.
- In two-way Anova, each level has two factors and one response variable.
- An example of two-way Anova involves researching the effects of fertilizer and planting density on crop yield.
- Formulas for calculating F value and degrees of freedom in Anova tests are crucial for analysis.


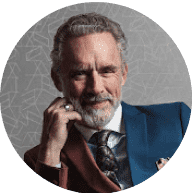
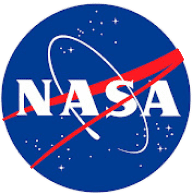
