ChatGPT: 30 Year History | How AI Learned to Talk
Art of the Problem・2 minutes read
Chachi BT created a groundbreaking computer program, leading to advancements in neural network research focusing on language processing and context understanding, culminating in the development of powerful AI models like GPT3 and Chat GPT with in-context learning and enhanced conversation capabilities. The evolution towards larger networks like GPT4 aims to unify AI research around language-based prediction systems, pushing the boundaries of intelligent agent capabilities.
Insights
- Chachi BT's groundbreaking computer program marked a significant leap in computer capabilities, showcasing human-like interaction possibilities previously unseen.
- The evolution from narrow task-focused neural networks to the revolutionary Transformer architecture with self-attention layers revolutionized text processing, enabling networks to consider all words simultaneously and generate contextually accurate text, paving the way for more advanced language-based prediction systems like GPT4.
Get key ideas from YouTube videos. It’s free
Recent questions
What is the significance of recurrent neural networks in language training?
Recurrent neural networks play a crucial role in language training by allowing networks to learn sequential patterns and understand word boundaries and meanings. Through experiments like Jordan's and Elman's, researchers have demonstrated the network's ability to predict sequential patterns and learn language structures. This ability mirrors how humans learn language, with sequences of words forming pathways in the network. The development of self-attention layers further enhanced the context understanding in text processing, enabling words to compare and absorb meaning from other words. Overall, recurrent neural networks have revolutionized language training by improving the network's ability to generate coherent and contextually accurate text.
How did Karpathy's experiment contribute to the advancement of neural networks?
Karpathy's experiment on training a larger network on Shakespeare and mathematics papers showcased the network's ability to learn and generate coherent text. By training the network on a diverse range of texts, Karpathy demonstrated the network's capacity to understand and produce language effectively. This experiment highlighted the potential of neural networks in text generation tasks and paved the way for further research in natural language processing. Karpathy's work contributed to the understanding of how neural networks can learn complex concepts and generate meaningful text, pushing the boundaries of what was previously thought possible in computer-generated language.
What led to the development of self-attention layers in neural networks?
The memory constraint in recurrent neural networks prompted the development of self-attention layers in neural networks. These layers were designed to improve context understanding in text processing by allowing words to compare and absorb meaning from other words. By enabling a more contextually aware network, self-attention layers addressed the limitations of traditional recurrent networks and enhanced the network's ability to process and generate coherent text. The introduction of self-attention layers marked a significant advancement in neural network architecture, leading to more sophisticated and effective text processing models.
How did GPT3 revolutionize language-based prediction systems?
GPT3 revolutionized language-based prediction systems by introducing in-context learning, where the network could learn new information without altering its weights. This new computing paradigm allowed the computer to respond to prompts at the thought level, enabling more dynamic and adaptive interactions. With 175 billion connections and 96 layers, GPT3 showed enhanced performance compared to its predecessors, showcasing the potential of large-scale language models in various tasks like translation and summarization. The development of GPT3 marked a significant milestone in AI research, pushing the boundaries of what neural networks could achieve in language processing.
What impact did Chat GPT have on AI experimentation and human conversations?
Chat GPT had a significant impact on AI experimentation and human conversations by enabling more effective interactions and sparking a wave of AI innovation. By training on human instructions and incorporating prompts like "think step by step," Chat GPT improved its performance in generating coherent and contextually accurate responses. This led to experiments in self-talk and the integration of language models into virtual and real-world tasks, expanding the applications of AI in various domains. With over 100 million users engaging with Chat GPT, the model demonstrated the potential of large-scale language models in enhancing human-computer interactions and driving advancements in AI technology.
Related videos
Andrej Karpathy
Let's build GPT: from scratch, in code, spelled out.
RationalAnswer | Павел Комаровский
Как работает ChatGPT: объясняем нейросети просто
Joshua Chang
NEW GPT-4o: My Mind is Blown.
The Royal Institution
What's the future for generative AI? - The Turing Lectures with Mike Wooldridge
CS50
GPT-4 - How does it work, and how do I build apps with it? - CS50 Tech Talk
Summary
00:00
Evolution of Neural Networks in Text Processing
- Chachi BT released the first widely available computer program allowing human-like interaction, surpassing previous beliefs in computer capabilities.
- The series explores the evolution of neural network research, initially focused on narrow tasks through supervised learning.
- Jordan's 1986 experiment trained a neural network to predict sequential patterns, introducing the concept of recurrent neural networks.
- Elman's experiment with language training on a neural network revealed the network's ability to learn word boundaries and meanings.
- Training neural networks by hiding the next event mirrors how humans learn language, with sequences of words forming pathways.
- Karpathy's experiment on a larger network, trained on Shakespeare and mathematics papers, demonstrated the network's ability to learn and generate coherent text.
- OpenAI's team in 2017 built a larger recurrent network trained on Amazon reviews, discovering neurons representing complex concepts like sentiment.
- The memory constraint in recurrent neural networks led to the development of self-attention layers, allowing for better context understanding in text processing.
- Self-attention layers enable words to compare and absorb meaning from other words, creating a more contextually aware network.
- The Transformer architecture, utilizing self-attention, revolutionized text processing by allowing networks to consider all words simultaneously, leading to more coherent and contextually accurate text generation.
16:55
Advancements in Language Models and AI
- Researchers experimented with language models, starting with GPT2, using a large web-scraped dataset and a network with 300,000 neurons, achieving impressive results in tasks like translation and summarization.
- Despite concerns about potential misuse, GPT2 struggled with coherence over extended periods, leading researchers to increase the network size by 100 times for GPT3, which had 175 billion connections and 96 layers, showing enhanced performance.
- GPT3 introduced in-context learning, allowing the network to learn new information without altering its weights, showcasing a new computing paradigm where the computer responds to prompts at the thought level.
- Further training on human instructions led to the development of Chat GPT, enabling more effective human conversations and sparking a wave of AI experimentation with over 100 million users.
- Adding "think step by step" to prompts significantly improved Chat GPT's performance, leading to experiments in self-talk and the integration of language models into virtual and real-world tasks.
- The progression towards larger networks like GPT4 aimed to create the most capable intelligent agent, potentially unifying AI research around a single direction focused on language-based prediction systems.


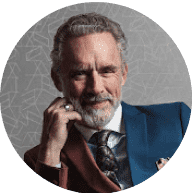
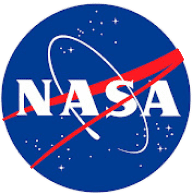
