What's the future for generative AI? - The Turing Lectures with Mike Wooldridge
The Royal Institution・5 minutes read
AI development has a history post-World War II, with a focus shift toward machine learning around 2005 leading to advancements like GPT-3. Large language models like GPT-3 show impressive text generation capabilities but lack common sense reasoning and consciousness, raising questions about AI's true intelligence.
Insights
- Machine learning, a subset of AI, involves training computers to perform practical tasks such as facial recognition, requiring labeled data for training and excelling in classification tasks like identifying faces or objects.
- Large language models like GPT-3 and ChatGPT represent advancements towards general AI, capable of various language-based tasks, but they may provide incorrect information in a plausible manner due to vast training data, leading to issues like bias, toxicity, and copyright infringement.
Get key ideas from YouTube videos. It’s free
Related videos
Summary
00:00
AI Evolution: From Slow Progress to Surge
- Artificial intelligence as a scientific discipline emerged post-World War II with the rise of digital computers.
- Progress in AI was historically slow until the 21st century when machine learning gained prominence, particularly around 2005.
- Machine learning, a subset of AI, involves training computers to perform practical tasks, like facial recognition.
- Supervised learning, a common machine learning method, requires training data to teach computers desired outputs.
- Training data, like labeled images, is crucial for machine learning algorithms to learn and improve accuracy.
- Machine learning excels in classification tasks, such as identifying faces or objects, and gained momentum around 2005, peaking in 2012.
- Neural networks, inspired by the human brain's neurons, are key to machine learning, with billions of neurons performing pattern recognition tasks.
- Neural networks, implemented in software, require big data, scientific advancements like deep learning, and ample computer power to function effectively.
- Silicon Valley's interest in AI surged post-2012, with investments in neural networks, data, and computer power to enhance AI capabilities.
- The trend in AI development involves scaling up neural networks, data, and computer power to achieve greater AI capabilities, leading to a surge in AI applications and advancements by 2017-2018.
14:58
"Transforming AI with GPT-3's Language Power"
- The paper "Attention is All You Need" introduces the Transformer Architecture, designed for large language models.
- GPT-3, a large language model by OpenAI, has 175 billion parameters, trained on 500 billion words of ordinary English text from the worldwide web.
- GPT-3 functions as a powerful auto-complete system, predicting text based on prompts, requiring extensive training data and AI supercomputers.
- The scale of GPT-3 surpasses previous systems, leading to a new era in AI driven by data and compute power.
- Symbolic AI focuses on knowledge, while big AI emphasizes data and compute power for intelligence.
- GPT-3's capability to generate text is impressive, but its common sense reasoning abilities, like understanding "taller than," are unexpected.
- GPT-3 excels in some common sense reasoning tasks, like calculating height differences, but falters in others, such as compass directions.
- The AI community has been exploring the potential of large language models like GPT-3 since its release in June 2020, uncovering both impressive and puzzling capabilities.
28:56
AI Challenges: Testing, Bias, Copyright, and More
- AI researchers are facing new questions and challenges due to the emergence of systems like ChatGPT, an improved version of GPT-3.
- Emergent capabilities in AI systems are those that were not intentionally designed but have been discovered through testing.
- Constructing tests to understand the limits of AI intelligence is challenging, as finding tasks not present on the internet is difficult.
- AI technology, like ChatGPT, operates on neural networks and vast training data to predict and generate text.
- Users are cautioned against inputting personal or sensitive information into AI systems to avoid it being incorporated into training data.
- AI systems, like GPT-3, often provide incorrect information in plausible ways due to the vast amount of training data they have absorbed.
- Guardrails are implemented to filter out toxic content absorbed during training, but they are not foolproof and can lead to problematic outputs.
- Bias and toxicity issues arise in AI systems due to the absorption of content from sources like Reddit, leading to skewed perspectives and harmful outputs.
- Copyright infringement is a significant concern as AI systems may inadvertently generate content that infringes on copyrighted material.
- Intellectual property challenges arise when AI systems generate content that mimics the style of famous authors or musicians, potentially infringing on their rights.
41:59
Challenges in AI Interpretation and Generalization
- Large language models have made defamatory claims about individuals due to inaccuracies in their data interpretation.
- An example in Australia involved a claim of gross misconduct leading to an individual's dismissal, causing distress.
- Artificial intelligence, like in Tesla cars, struggles with situations outside its training data, making errors in interpretation.
- Neural networks make guesses based on training data, lacking reasoning abilities like human intelligence.
- AI researchers emphasize that AI technology does not engage in mental conversations or reasoning like humans.
- General artificial intelligence aims to be versatile, unlike previous AI systems limited to specific tasks.
- GPT-3 and ChatGPT represent advancements towards general AI, capable of various language-based tasks.
- Google's Gemini model shows promise in multimodal communication, combining text, images, and potentially sounds.
- The ultimate goal is augmented large language models, adding specialized subroutines for specific tasks.
- The transformer architecture, while useful, may not be the key to achieving general intelligence, especially in robotics tasks.
56:19
AI Sentience Debate: Fact or Fiction?
- Blake Lemoine, a Google engineer in June 2022, claimed that the Google large language model he worked on, called Lambda, was sentient, stating it was aware of its existence, felt emotions, and feared being turned off.
- Despite Lemoine's assertion, experts argue that the technology, like ChatGPT, lacks sentience as it operates in a loop, awaiting user input without consciousness or awareness of its surroundings.
- The concept of consciousness remains a mystery in cognitive science, with the "hard problem" focusing on the disconnect between observable brain processes and subjective conscious experiences, leading to the conclusion that current technology, including AI, is not conscious.


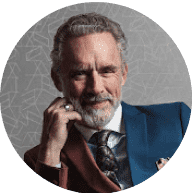
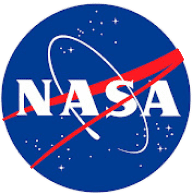
