Statistics 101: Understanding Correlation
Brandon Foltz・19 minutes read
The video series explains bivariate relationships in statistics, focusing on correlation and its distinction from covariance, while illustrating concepts with real-world examples like the relationship between the S&P 500 and Dow Jones. It emphasizes that correlation measures both the strength and direction of a relationship, is standardized, and warns against assuming causation from correlation without analyzing scatterplots.
Insights
- The video series focuses on the concept of correlation in statistics, explaining that while covariance shows how two variables vary together, correlation provides a standardized measure of both the strength and direction of their relationship, making it more useful for comparisons across different scales. The speaker highlights the importance of examining scatterplots to ensure a linear relationship exists before calculating correlation, cautioning that correlation does not imply causation and providing practical examples, such as the strong correlation between the S&P 500 and Dow Jones indices.
- Rising Hills Manufacturing's study illustrates the application of these concepts, as they calculated a strong positive correlation of 0.989 between the number of workers and tables produced, demonstrating a significant linear relationship. The video also notes a rule of thumb for assessing relationships, stating that a correlation coefficient exceeding 0.632 for a sample size of 10 indicates a relationship, emphasizing the need for careful analysis when interpreting statistical data.
Get key ideas from YouTube videos. It’s free
Recent questions
What is correlation in statistics?
Correlation in statistics refers to a measure that indicates the strength and direction of a linear relationship between two variables. It is quantified using the correlation coefficient, often denoted as "r," which ranges from -1 to +1. A value of 1 indicates a perfect positive correlation, meaning that as one variable increases, the other also increases proportionally. Conversely, a value of -1 indicates a perfect negative correlation, where one variable increases as the other decreases. A correlation of 0 suggests no linear relationship between the variables. Understanding correlation is essential for analyzing data, as it helps in identifying patterns and making predictions based on the relationship between different factors.
How to improve in statistics?
Improving in statistics requires a combination of practice, understanding fundamental concepts, and maintaining a positive mindset. One effective approach is to engage with various resources, such as textbooks, online courses, and video tutorials, which can provide different perspectives on complex topics. Regularly practicing problems, especially those involving real-world data, can enhance your skills and confidence. Additionally, collaborating with peers or seeking help from instructors can clarify difficult concepts. It's important to remember that mastery in statistics, like any other subject, comes with time and effort, so staying motivated and persistent is key to improvement.
What is covariance in statistics?
Covariance is a statistical measure that indicates the extent to which two variables change together. It provides insight into the direction of the relationship between the variables: a positive covariance suggests that as one variable increases, the other tends to increase as well, while a negative covariance indicates that as one variable increases, the other tends to decrease. However, covariance does not provide a standardized measure, meaning its value can vary significantly depending on the scale of the variables involved. This makes it less interpretable compared to correlation, which standardizes the relationship between variables, allowing for easier comparison across different datasets.
What does a scatterplot show?
A scatterplot is a graphical representation that displays the relationship between two quantitative variables. Each point on the scatterplot corresponds to an observation in the dataset, with one variable plotted along the x-axis and the other along the y-axis. By examining the pattern of points, one can identify the nature of the relationship—whether it is positive, negative, or non-linear. Scatterplots are particularly useful for visualizing correlations, as they allow for a quick assessment of how closely the data points cluster around a line, indicating the strength of the relationship. They also help in identifying outliers and understanding the overall distribution of the data.
Does correlation imply causation?
Correlation does not imply causation, which is a common misconception in statistics. While correlation indicates a relationship between two variables, it does not provide evidence that one variable causes changes in the other. There are several reasons why two variables may be correlated, including the possibility of a third variable influencing both, or the correlation being purely coincidental. Therefore, it is crucial to conduct further analysis, such as controlled experiments or additional statistical tests, to establish a causal relationship. Understanding this distinction is vital for accurate data interpretation and avoiding erroneous conclusions in research and analysis.
Related videos
Tech Classes
Complete STATISTICS for Data Science | Data Analysis | Full Crash Course
Brandon Foltz
Statistics 101: Linear Regression, Algebra, Equations, and Patterns
Ace Maths
KS3 Maths - Scatter Graphs
Catholic University MPP Program
Lecture 3
Khan Academy
Statistical questions | Data and statistics | 6th grade | Khan Academy
Summary
00:00
Understanding Correlation in Bivariate Statistics
- The video series focuses on basic statistics, specifically on bivariate relationships, with this installment dedicated to understanding correlation, following a previous discussion on covariance.
- The speaker encourages viewers who may be struggling in their statistics classes to remain positive, emphasizing that hard work and practice can lead to improvement, and invites them to follow him on YouTube and Twitter for updates on new content.
- A scatterplot of monthly returns for the S&P 500 and Dow Jones Industrial Average in 2012 is presented, illustrating a linear pattern where both indices tend to rise and fall together, indicating a positive linear relationship.
- Covariance is defined as a measure of how two variables vary together, while correlation is introduced as a more comprehensive measure that indicates both the direction and strength of the relationship between two variables.
- The covariance lacks upper or lower boundaries and is dependent on the scale of the variables, whereas correlation is standardized, ranging from -1 to +1, allowing for comparisons across different measurement scales.
- The speaker stresses the importance of examining scatterplots before calculating correlations, as correlation is only applicable to linear relationships, and warns against the misconception that correlation implies causation.
- Examples of non-linear relationships are provided, including a U-shaped relationship between energy usage and temperature, highlighting that correlation is not suitable for such data patterns.
- The correlation coefficient, denoted as "r," is introduced as the Pearson correlation coefficient, calculated by dividing the covariance of two variables by the product of their standard deviations, providing a standardized measure of their relationship.
- A practical example is given where the correlation between the S&P 500 and Dow Jones is calculated using statistical software, yielding a correlation of 0.974, indicating a strong positive relationship.
- The video concludes with a brief overview of the correlation formula, emphasizing its components and the significance of understanding the relationship between covariance and standard deviations in calculating correlation.
17:11
Correlation Analysis of Workers and Production
- Rising Hills Manufacturing conducted a study to analyze the relationship between the number of workers (x) and the number of tables produced (y) using 10 one-hour samples from the production floor, with standard deviations of 6.48 for workers and 16.69 for tables produced.
- The covariance between the number of workers and tables produced was calculated as 106.93, derived from the formula s_xy = 962.4 / (n - 1), where n is the number of samples.
- The correlation coefficient (r) was computed using the formula r = covariance / (standard deviation of x * standard deviation of y), resulting in a strong positive correlation of 0.989, indicating a significant linear relationship between the two variables.
- A rule of thumb for determining the existence of a relationship between two variables states that if the absolute value of the correlation coefficient exceeds 2 divided by the square root of the sample size (0.632 for 10 samples), a relationship is considered to exist.
- Covariance indicates the direction of the relationship but lacks a standardized scale, while correlation is bounded between -1 and 1, providing both direction and strength of the relationship, making it a more interpretable measure.
- The video emphasizes that correlation does not imply causation, and it is crucial to examine scatterplots to confirm the linear relationship before drawing conclusions about the relationship between the variables.


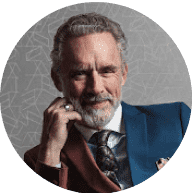
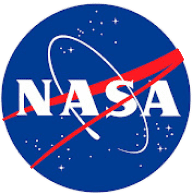
