Statistics 101: Linear Regression, Algebra, Equations, and Patterns
Brandon Foltz・4 minutes read
The video provides an introduction to simple linear regression for beginners, emphasizing the importance of a positive mindset and community support in overcoming statistical challenges. It explains the relationship between dependent and independent variables, illustrates key concepts with example equations, and outlines the next steps for analyzing the relationship between bill amounts and tips.
Insights
- The video serves as an introductory resource for those new to statistics, particularly focusing on simple linear regression, and aims to create a positive learning atmosphere by encouraging viewers to persist through challenges, as highlighted by the instructor's assurance that dedication and patience can lead to success in statistics.
- Simple linear regression is explained as a method to understand the relationship between two variables, where the dependent variable (y) is influenced by the independent variable (x), illustrated through various regression equations that demonstrate how the slope and y-intercept define the nature of this relationship, ultimately guiding viewers to explore real-world applications such as predicting tip amounts based on bill amounts.
Get key ideas from YouTube videos. It’s free
Recent questions
What is simple linear regression?
Simple linear regression is a statistical method used to model the relationship between two variables by fitting a linear equation to observed data. In this context, one variable is considered the independent variable (x), while the other is the dependent variable (y). The goal is to find the best-fitting line that describes how changes in the independent variable affect the dependent variable. This relationship is typically expressed in the slope-intercept form of a line, y = mx + b, where 'm' represents the slope and 'b' is the y-intercept. Simple linear regression is foundational in statistics and is often used in various fields to make predictions and understand relationships between variables.
How do I calculate the slope of a line?
The slope of a line in the context of linear regression is calculated by determining the change in the dependent variable (y) for a one-unit change in the independent variable (x). Mathematically, the slope (m) can be derived from the regression equation, which is often expressed as y = mx + b. For example, if the regression equation is y = 2x + 3, the slope is 2, indicating that for every increase of 1 unit in x, y increases by 2 units. The slope is a crucial component of the regression line, as it provides insight into the strength and direction of the relationship between the two variables being analyzed.
What is the purpose of regression analysis?
The purpose of regression analysis is to understand and quantify the relationship between one or more independent variables and a dependent variable. It allows researchers and analysts to make predictions, assess the strength of relationships, and identify trends within data. By fitting a regression model to the data, one can estimate how changes in the independent variable(s) influence the dependent variable. This analysis is widely used in various fields, including economics, biology, and social sciences, to inform decision-making, test hypotheses, and guide future research. Ultimately, regression analysis provides a powerful tool for interpreting complex data and drawing meaningful conclusions.
What is a residual in regression?
A residual in regression analysis is the difference between the observed value of the dependent variable and the value predicted by the regression model. It is calculated as the actual value minus the predicted value (Residual = Actual - Predicted). Residuals are important because they provide insight into the accuracy of the regression model; smaller residuals indicate a better fit of the model to the data. Analyzing residuals can help identify patterns that suggest the model may not adequately capture the relationship between the variables, leading to potential improvements in the model. Understanding residuals is crucial for assessing the performance of regression models and ensuring reliable predictions.
How do I interpret the y-intercept in regression?
The y-intercept in regression analysis represents the expected value of the dependent variable when the independent variable is equal to zero. In the slope-intercept form of a linear equation, y = mx + b, 'b' is the y-intercept. For example, if the regression equation is y = 5 + 2x, the y-intercept is 5, indicating that when x is zero, the expected value of y is 5. The y-intercept provides a baseline for understanding the relationship between the variables and can be particularly meaningful in contexts where the independent variable can logically take on a value of zero. However, it is essential to consider the context of the data, as the y-intercept may not always have a practical interpretation if a zero value for the independent variable is not realistic.
Related videos
Summary
00:00
Understanding Simple Linear Regression Basics
- The video is part of a basic statistics series focusing on simple linear regression, aimed at individuals new to the subject, and emphasizes a supportive learning environment for those struggling with statistics.
- The instructor encourages viewers to stay positive and assures them that with hard work and patience, they can overcome challenges in their statistics class.
- Viewers are invited to connect with the instructor on social media platforms like YouTube, Twitter, Google+, and LinkedIn to stay updated on new content and foster community engagement.
- The video builds on previous discussions about regression, residuals, and the sum of squares, aiming to introduce essential terminology and concepts related to regression analysis.
- Simple linear regression is categorized under bivariate statistics, which involves two variables, and shares characteristics with correlation and ANOVA, both of which also utilize scatter plots for data representation.
- The relationship in regression is defined as the dependent variable (y) being a function of the independent variable (x), expressed mathematically as y = f(x).
- The slope-intercept form of a line, y = mx + b, is crucial for understanding regression lines, where 'm' represents the slope and 'b' is the y-intercept, indicating where the line crosses the y-axis.
- An example equation, y = 2x + 3, illustrates how to identify the slope (2) and y-intercept (3), with the slope indicating a rise of 2 units for every 1 unit run along the x-axis.
- The overall regression model for a population is expressed as y = β₀ + β₁x + e, where β₀ is the y-intercept, β₁ is the slope, and 'e' represents the error term, indicating unexplained variation in the dependent variable.
- The expected value of y in regression is the mean of a distribution of y values for a given x, and regression lines can take three forms: a flat line (slope = 0), a positively sloped line, or a negatively sloped line, each representing different relationships between the variables.
15:35
Understanding Linear Regression and Its Implications
- The expected value of y in a linear regression is represented by the equation y = β₀ - β₁x, where β₁ indicates the slope; a negative β₁ results in a line descending from the top left to the bottom right, while a positive β₁ results in an ascending line from the bottom left to the top right.
- When using sample data instead of population parameters, the regression equation is modified to y-hat = b₀ + b₁x, where y-hat is the point estimator of the expected value of y, and lowercase b replaces the population parameters β.
- In a scenario where only the dependent variable (tip amount) is available, the mean tip amount was calculated to be $10, leading to a residual sum of squares of 120, with the slope of the regression line being zero since no independent variable was present.
- The regression model is always compared to a situation where the slope is zero, meaning y-hat equals 10 for every value of x, indicating that the expected tip amount remains constant regardless of the bill amount.
- Three example regression equations were analyzed: y-hat = 0.3 - 3.3x (negative slope), y-hat = 48 + 7.8x (positive slope), and y-hat = 14.87 - 0.014x (slope close to zero), illustrating how the sign and value of the slope affect the graph's appearance.
- The next steps involve adding actual bill amounts to the tip data, hypothesizing a linear relationship where higher bills lead to higher tips, and determining the effectiveness of the regression model by comparing the residual sum of squares from the regression line to that of the mean tip amount.


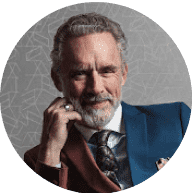
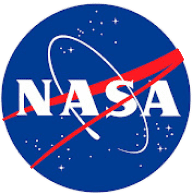
