What is a Data Model?
ness-intricity101γ»2 minutes read
A data model is crucial for establishing relationships within a database and requires collaboration between business stakeholders and data architects to accurately reflect specific needs; however, organizations often mistakenly rely on packaged data models, which may lead to significant misalignments and costly modifications. To avoid these pitfalls, it's essential to thoroughly vet packaged models and consider customizing them to incorporate unique business practices, potentially leveraging expert guidance for effective implementation.
Insights
- A data model is crucial for establishing relationships within a database and requires collaboration between business stakeholders and data architects to ensure it effectively addresses specific organizational needs. Relying on packaged data models can be misleading, as they often necessitate extensive vetting and may not align with an organization's unique practices, leading to costly modifications during implementation.
- To prevent issues with reporting and analytics, organizations should carefully evaluate any packaged data model and consider it a starting point rather than a complete solution. Customizing the model to fit unique business processes is essential, and seeking expert advice, such as from Intricity, can enhance the implementation strategy and provide long-term advantages.
Get key ideas from YouTube videos. Itβs free
Recent questions
What is a data model?
A data model is a conceptual framework that defines how data is organized, structured, and related within a database. It serves as a blueprint for data management and is crucial for data warehousing, ensuring that data can be efficiently stored, retrieved, and analyzed. By outlining the relationships between different data elements, a data model helps organizations understand their data landscape and supports effective decision-making. It is essential for aligning data architecture with business needs, making it a foundational component in the development of robust data systems.
How to choose a data model?
Choosing a data model involves several critical steps to ensure it aligns with an organization's specific needs and practices. First, it is important to engage business stakeholders in discussions to gather requirements and understand the unique aspects of the business. This collaborative approach helps in identifying the necessary data elements and relationships. Organizations often consider packaged data models for convenience, but it is vital to thoroughly vet these models to ensure they fit the internal processes. Customizing a data model to reflect the organization's practices can lead to better outcomes, so seeking expert advice can also be beneficial in making an informed choice.
Why are packaged data models risky?
Packaged data models can be risky because they may not align with an organization's internal practices and requirements. When organizations implement these models without proper vetting, they often encounter significant issues during reporting and analytics phases. Common problems include missing data dimensions or misaligned logic, which can lead to costly modifications and disruptions. This situation is akin to a poorly executed home renovation, where foundational issues necessitate extensive rework. To mitigate these risks, organizations should treat packaged models as starting points and customize them to fit their unique needs, ensuring a smoother implementation process.
What are the benefits of a custom data model?
A custom data model offers numerous benefits tailored to an organization's specific needs and competitive advantages. By developing a model that reflects unique business practices, organizations can ensure that their data architecture supports effective reporting and analytics. Custom models allow for the incorporation of relevant data dimensions and relationships, leading to more accurate insights and decision-making. Additionally, a well-designed custom data model can enhance data quality and integrity, ultimately resulting in long-term benefits such as improved operational efficiency and better alignment with strategic goals. Engaging experts in the process can further enhance the effectiveness of the model.
How to implement a data model effectively?
Implementing a data model effectively requires a structured approach that includes thorough planning, stakeholder engagement, and continuous evaluation. Initially, organizations should involve business stakeholders in facilitated sessions to gather insights and requirements, ensuring the model aligns with their needs. Once a model is selected or customized, it is crucial to conduct rigorous testing to identify any potential issues before full deployment. Organizations should also establish clear processes for data integration, ETL (Extract, Transform, Load), and reporting to ensure seamless functionality. Seeking expert guidance can provide valuable strategies and insights, ultimately leading to a successful implementation that maximizes the model's benefits.
Related videos
Summary
00:00
Navigating the Challenges of Data Models
- A data model serves as the framework for relationships within a database, essential for data warehousing, and requires active participation from business stakeholders in facilitated sessions with data architects to ensure it meets specific needs. Organizations often seek out packaged data models, mistakenly believing they will save time and effort, but the vetting process for these models can be as labor-intensive as creating a custom model tailored to the business.
- The primary challenge with packaged data models is that they often do not align with an organization's internal practices, leading to significant issues once reporting and analytics are implemented. Organizations may discover missing dimensions or misaligned logic, resulting in costly modifications akin to a home renovation where plumbing is incorrectly installed, necessitating a complete overhaul of the data warehouse components, including ETL, staging, and reports.
- To avoid pitfalls, organizations should thoroughly vet any packaged data model before deployment, treating it as a guide rather than a final solution. Customizing the model allows for the incorporation of unique business practices and competitive advantages, and seeking expert guidance, such as from Intricity, can provide valuable insights and strategies for effective data model implementation, ultimately yielding substantial long-term benefits.


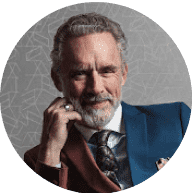
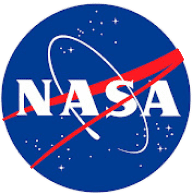
