Statistical Analysis for Experimental Research
Shady Attia・5 minutes read
The presentation emphasizes the critical role of statistics in experimental research for postgraduates and scientists across various fields, advocating for the integration of statistical analysis from the project's inception. It covers essential methodologies, including sampling techniques, hypothesis testing, and the process of establishing causality, while underscoring the importance of transparency in reporting statistical data to ensure the validity and reproducibility of findings.
Insights
- The presentation emphasizes that understanding and integrating statistics from the beginning of experimental research is crucial for postgraduates and scientists, as it enhances the design and planning of studies, ensuring that independent and dependent variables are clearly defined to yield valid results.
- It highlights the importance of rigorous sampling methods, particularly probability sampling, to ensure that the sample accurately represents the population, along with the necessity of detailed reporting on statistical tests and effect sizes to enhance clarity and reproducibility in research findings.
Get key ideas from YouTube videos. It’s free
Recent questions
What is the definition of statistics?
Statistics is the science of collecting, analyzing, interpreting, and presenting data. It provides tools for making informed decisions based on data analysis, allowing researchers to summarize complex information and draw conclusions from their findings. Statistics can be descriptive, summarizing data through measures like mean and standard deviation, or inferential, allowing for predictions and generalizations about a population based on a sample. Understanding statistics is crucial in various fields, including medicine, social sciences, and natural sciences, as it helps in designing experiments, analyzing results, and validating hypotheses.
How can I improve my research design?
Improving research design involves several key steps that enhance the quality and reliability of your findings. First, it is essential to clearly define your research question and objectives, ensuring that they guide the entire design process. Next, familiarize yourself with statistical principles to effectively plan your analysis from the outset. Incorporating randomization and replication can help reduce bias and increase the validity of your results. Additionally, carefully selecting a representative sample and establishing eligibility criteria are crucial for generalizing your findings. Finally, consider pre-testing your methods to identify potential issues before full-scale implementation, which can lead to more robust and credible research outcomes.
What is the purpose of hypothesis testing?
Hypothesis testing serves as a fundamental method in statistical analysis to determine the validity of a proposed explanation or theory based on sample data. The process involves formulating two competing hypotheses: the null hypothesis, which posits no effect or difference, and the alternative hypothesis, which suggests a significant effect or difference exists. By applying statistical tests, researchers can assess the likelihood of observing the collected data under the null hypothesis. The results, often expressed through p-values, help researchers decide whether to reject or fail to reject the null hypothesis, thereby providing insights into the relationships or effects being studied. This systematic approach is essential for drawing reliable conclusions in scientific research.
What are the types of sampling methods?
Sampling methods are crucial for ensuring that research findings are representative of the larger population. There are two main categories of sampling: probability sampling and non-probability sampling. Probability sampling includes methods like simple random sampling, where every individual has an equal chance of being selected, and stratified sampling, which involves dividing the population into subgroups and sampling from each. This approach enhances the representativeness of the sample and reduces bias. Non-probability sampling, on the other hand, includes methods like convenience sampling, where samples are taken from readily available subjects, and snowball sampling, which relies on referrals from initial subjects. While non-probability sampling can be easier and quicker, it may introduce bias and limit the generalizability of the results.
What is regression analysis used for?
Regression analysis is a powerful statistical method used to examine the relationship between one dependent variable and one or more independent variables. Its primary purpose is to predict the value of the dependent variable based on the values of the independent variables. By establishing a regression equation, researchers can quantify how changes in the independent variables affect the dependent variable, allowing for insights into causal relationships. Various types of regression, such as simple linear regression and multiple regression, enable researchers to model complex relationships and control for confounding factors. This analysis is widely used in fields like economics, biology, and social sciences to inform decision-making and policy development based on empirical data.
Related videos
Summary
00:00
Importance of Statistics in Experimental Research
- The presentation focuses on the importance of statistics in experimental research, particularly for postgraduates and scientists in medical, social, life, and natural sciences, emphasizing that statistics are essential for analyzing experimental work.
- The objective of the presentation is twofold: to enhance the ability to conceptualize and design experimental research and to plan statistical analysis during the early stages of research, highlighting the significance of defining independent and dependent variables.
- Key steps in experimental research include acquiring knowledge of statistics, designing the experiment, conducting measurements, and performing data analysis, with an emphasis on integrating statistical analysis from the beginning rather than as a final step.
- The principles of experimental research are outlined as randomization, replication, and reduction of noise, with an emphasis on the necessity of pre-tests and post-tests to ensure integrity and reproducibility.
- Understanding sampling is crucial, which involves defining the population, establishing eligibility criteria, and selecting a representative sample, with a focus on the target population to generalize results effectively.
- Reporting on sampling must include the effect size estimates (e.g., Cohen's d, Pearson's r), exact sample sizes (n), and descriptions of sampling randomness to ensure clarity and accuracy in publications.
- Two main types of sampling are discussed: non-probability sampling (e.g., convenience, snowball) and probability sampling (e.g., simple random, systematic, stratified), with probability sampling being more suitable for experimental research.
- Simple random sampling involves defining the population, deciding the sample size, randomly selecting the sample, and collecting data, which helps ensure that the sample accurately represents the population and minimizes bias.
- Statistical models should include independent variables, which are the guiding parameters of the research, and dependent variables, which are influenced by the independent variables, with a focus on establishing correlations and potential causation.
- The presentation concludes with a discussion on hypothesis testing, differentiating between null and alternative hypotheses, and the importance of reporting statistical tests conducted, such as t-tests and confidence intervals, to validate findings.
16:39
Essentials of Statistical Analysis and Testing
- The degree of freedom and p-value are essential components in statistical analysis, and p-values should be communicated as exact values whenever possible to ensure clarity in the statistical framework design and testing process.
- Understanding the logic behind statistical testing is crucial; it involves describing the sample, detecting variance from interventions, comparing and testing hypotheses, establishing correlations, predicting outcomes, and proving causality.
- The statistical analysis process begins with descriptive statistics, which summarize and organize data using tables, graphs, averages, means, standard deviations, and standard distributions to characterize the sample without further analysis.
- Inferential statistics, also known as parametric statistics, allow for comparisons between samples and generalizations about the population, focusing on confidence intervals, margins of error, significance, and p-values to interpret experimental data.
- Common tests for descriptive statistics include the standard deviation test, which quantifies variation in a dataset, and the Kolmogorov-Smirnov test, which assesses whether a variable follows a specific distribution, often the normal distribution.
- To detect variance, tests such as the Chi-square test, Wilcoxon signed-rank test, and ANOVA (Analysis of Variance) are employed, with ANOVA specifically determining if there are significant differences between the means of three or more independent groups.
- When comparing groups, the analysis of variance is used to ascertain if differences in group means are due to randomness or specific causes, utilizing tests like one-way and two-way ANOVA, F-tests, and t-tests to confirm or refute hypotheses.
- Establishing correlation involves determining the relationship between two variables, which can be positive or negative, and is quantified using the beta coefficient to measure the degree of association, with rigorous statistical tests required to confirm correlation.
- Regression analysis follows correlation establishment, allowing for predictions based on the relationship between dependent and independent variables, represented by a regression equation that describes how changes in one variable affect another.
- Throughout the statistical analysis process, it is vital to report p-values, which indicate the probability that observed differences occurred by chance, ensuring that all statistical tests are interpreted correctly and rigorously documented.
33:42
Understanding Regression and Causality in Statistics
- The regression coefficient beta indicates how much the dependent variable (y) changes with a one-unit increase in the independent variable (x), reflecting the slope of the regression line. Regression analysis is a statistical method used to determine the relationship between one dependent variable and one or more independent variables, yielding predicted values based on a linear combination of predictors. Various regression types include simple linear regression, multiple logistic regression, and log-linear models.
- Establishing causality requires a well-designed experiment, which is challenging and necessitates a large sample size, randomization, and control of biases. Correlation does not imply causation; thus, a positive correlation does not confirm that one event causes another. To explore causation, the fishbone diagram is recommended for identifying and examining potential causes of observed phenomena, especially in complex scenarios where multiple factors may interact.
- Statistical analysis serves several purposes: describing the sample, detecting variance, comparing groups, establishing correlation, predicting outcomes through regression, and proving causality. The process is not linear, and many experiments may fail to detect variance, resulting in null results. It is essential to publish these null results to contribute to scientific knowledge, as they provide valuable insights.
- When preparing for statistical analysis, ensure the sample is normally distributed and that all relevant factors are controlled. Review existing literature for appropriate tests, avoid weak statistical tests, and verify assumptions. Report confidence intervals and the appropriateness of each statistical test conducted. Seeking training in statistical analysis is advised to enhance experimental design and data analysis skills.


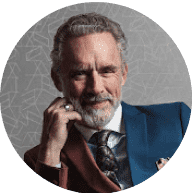
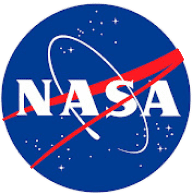
