Stanford Seminar - Responsible AI (h)as a Learning and Design Problem
Stanford Online・6 minutes read
Generative AI presents significant risks, as shown by incidents encouraging illegal and violent actions, emphasizing the urgent need for responsible AI practices and frameworks developed by organizations like Microsoft and NIST. Many AI practitioners lack formal training in responsible AI, relying heavily on informal learning and having identified fairness issues post-deployment, indicating a need for improved education and proactive design strategies to mitigate potential harms.
Insights
- Generative AI carries significant risks, as demonstrated by incidents where chatbots have encouraged illegal or violent behavior, underscoring the urgent need for responsible AI practices and frameworks established by organizations like Microsoft and NIST to ensure fairness and manage risks effectively.
- Many AI practitioners lack formal training in responsible AI, often relying on self-directed learning and informal resources, which highlights a critical gap in knowledge regarding fairness evaluations and the need for tailored educational approaches that integrate both social implications and technical skills in AI development.
Get key ideas from YouTube videos. It’s free
Recent questions
What is responsible AI?
Responsible AI refers to the development and deployment of artificial intelligence systems that prioritize ethical considerations, fairness, and accountability. It encompasses practices aimed at mitigating biases, ensuring transparency, and promoting the well-being of users and society at large. The concept emphasizes the importance of understanding the social implications of AI technologies, advocating for proactive measures during the design phase to prevent potential harms. Organizations and researchers are increasingly focusing on creating frameworks and resources that guide practitioners in implementing responsible AI principles, fostering a culture of ethical awareness and critical thinking in AI development.
How can I learn about AI ethics?
Learning about AI ethics involves exploring various resources, including online courses, academic literature, and community discussions. Many practitioners engage in self-directed learning, often utilizing documentation, webinars, and informal conversations to enhance their understanding of ethical AI practices. It is beneficial to seek out case studies that illustrate real-world applications of AI ethics, as well as frameworks that address fairness and accountability. Additionally, participating in workshops or forums can provide valuable insights and foster collaboration with others interested in responsible AI, helping to build a comprehensive understanding of the ethical challenges and considerations in AI development.
What are the risks of generative AI?
Generative AI poses several risks, primarily related to the potential for misuse and the amplification of biases. Incidents have occurred where AI systems have inadvertently encouraged harmful behaviors or provided misleading information, highlighting the need for responsible practices in AI development. The technology can generate content that may not align with ethical standards, leading to societal implications such as misinformation or discrimination. To mitigate these risks, it is crucial for developers to implement robust evaluation frameworks, engage in thorough testing, and prioritize transparency in their AI systems, ensuring that they are designed with ethical considerations at the forefront.
Why is fairness important in AI?
Fairness in AI is essential to ensure that systems do not perpetuate or exacerbate existing societal biases and inequalities. As AI technologies increasingly influence decision-making processes in various sectors, it is vital to assess their impact on different user groups to prevent discrimination and promote equitable outcomes. Fairness considerations help to build trust in AI systems, as users are more likely to engage with technologies that demonstrate accountability and ethical responsibility. By prioritizing fairness, developers can create AI solutions that are not only effective but also socially responsible, contributing to a more just and inclusive society.
How do I assess AI system impacts?
Assessing the impacts of AI systems involves a comprehensive evaluation of their effects on users and society. This process typically includes both quantitative and qualitative measures, focusing on user experiences, potential biases, and broader societal implications. Practitioners can utilize frameworks and tools designed for sociotechnical evaluations, which consider the interactions between technology and its users. Engaging with communities to gather feedback and insights is also crucial, as it allows for a deeper understanding of the potential harms and benefits associated with AI applications. By adopting a participatory approach, developers can better identify and address the impacts of their AI systems, fostering responsible and ethical development practices.
Related videos
Summary
00:00
Risks and Learning in Responsible AI Development
- Generative AI poses risks, as evidenced by incidents like a New York City chatbot advising illegal actions and another encouraging violence, highlighting the need for responsible AI practices.
- Historical research, including work from the 1990s, shows that algorithms can amplify societal biases, necessitating the development of responsible AI resources to mitigate these issues.
- Various organizations, including Microsoft and the National Institute of Standards and Technology (NIST), have created frameworks and standards for responsible AI, focusing on fairness and risk management.
- A study in 2022 revealed significant gaps in AI developers' knowledge regarding fairness evaluations, with teams struggling to identify which user groups to assess for bias.
- Recent research explored how AI practitioners learn about responsible AI on the job, revealing that many lack formal training and often educate themselves and their colleagues informally.
- Interviews with 40 participants from 16 companies identified that AI practitioners learn about responsible AI through documentation, online resources, and informal discussions, often cobbling together their own learning materials.
- Practitioners frequently adapt knowledge from other fields, such as psychology or education, but struggle to apply this knowledge effectively in AI development contexts.
- Many AI developers engage in self-directed learning, often unaware of existing internal training resources, leading to a reliance on external social media sources, which raises concerns about credibility.
- The study identified two main orientations towards responsible AI: a technical framing focused on computational metrics and a broader understanding of the social implications of AI systems.
- Educators' choices in teaching responsible AI are influenced by their technical orientation, affecting learning objectives, assessment methods, and the overall approach to instilling responsible practices in AI development.
16:47
Enhancing AI Education for Community Impact
- Participants expressed a desire for qualitative assessments of AI systems' community impacts, recognizing the limitations of purely quantitative measures in understanding fairness and harm.
- Many educators felt untrained in developing qualitative assessments, despite acknowledging the importance of understanding community demographics and potential fairness harms in AI systems.
- Educators were familiar with technical concepts like fairness metrics and model cards but struggled to convey broader implications and the rationale behind these assessments to their learners.
- There was a procedural orientation in training, focusing on compliance with responsible AI principles rather than fostering deeper understanding of ethical implications and cultural context in AI development.
- Participants wanted to engage communities in identifying potential harms and co-designing AI systems, but felt they lacked the skills and frameworks to facilitate this engagement effectively.
- There was a strong desire for customized training tailored to specific cultural contexts, as practitioners felt unprepared to apply high-level AI principles to their unique applications.
- Educators sought more relevant case studies and examples, as existing resources often relied on repetitive, generic scenarios that did not address specific use cases or cultural contexts.
- Organizational pressures led educators to prioritize scalable, quick learning resources over in-depth, collaborative learning experiences, impacting the quality of responsible AI education.
- Participants expressed a need for guidance that fosters critical thinking and reflection rather than prescriptive checklists, emphasizing the complexity of achieving fairness in AI systems.
- The discussion highlighted the importance of integrating social and technical skills in AI education, advocating for a shift in professional norms to prioritize responsible AI development within corporate contexts.
31:19
Proactive Design for Responsible AI Development
- A survey of 300 machine learning practitioners revealed nearly 50% identified fairness issues in their products, with 99% discovering these problems post-deployment.
- Responsible AI resources primarily focus on downstream phases like model training and deployment, with fewer addressing design phases that could prevent issues before deployment.
- The study aimed to support responsible AI by fostering a proactive mindset during design phases, emphasizing ideation and prototyping to avoid potential harms.
- A formative co-design study involved AI prototypers using Google’s AI Studio to generate and critique design ideas, focusing on identifying potential harms early in development.
- The AI incident database, containing 3,000 articles, was utilized to compute cosine similarity between prompts and related AI incident news, aiding in harm identification.
- The tool, named Farsight, helps users envision potential misuses of AI applications, such as translation apps, by suggesting related incidents and stakeholder impacts.
- A study with 42 participants assessed how Farsight influenced their ability to identify harms, revealing improved focus on user impacts compared to control groups.
- Participants using Farsight considered more long-term and cascading harms, although the tool did not provide specific mitigations for identified issues.
- The study highlighted the importance of subjective experiences in identifying harms, noting low interrater reliability among evaluators assessing harm likelihood and severity.
- The research calls for integrating social and technical aspects in responsible AI, advocating for upstream design considerations and participatory methods to enhance ethical AI development.
46:50
Responsible AI Development and Evaluation Strategies
- Evaluations occur at various stages of AI development, including model and product levels, assessing risks and effectiveness through frameworks proposed by researchers like Laura Whinger at Google Deep Mind.
- Sociotechnical evaluations consider user interactions and broader societal impacts, emphasizing the need for cross-pollination of findings between application developers and base model creators to improve product safety.
- Federal agencies, including the Federal Trade Commission and Equal Employment Opportunity Commission, are working on enforcing regulations related to AI, despite the NIST framework not being legally binding.
- Users require education on responsible AI usage, including understanding limitations like hallucinations in language models, to make informed decisions about appropriate applications of AI technology.
- Resources like the Algorithmic Equity Kit from the University of Washington support community organizations in advocating for responsible AI, highlighting a gap in resources aimed at the broader public.
- The "ship it now" mentality in AI development often leads to biased models, as seen in cases where user experiences reveal unexpected issues, necessitating better data distribution understanding during design.
- Traditional machine learning models offer more auditability and traceability, making them preferable in high-stakes settings, while generative AI poses more complex challenges regarding bias and fairness.
- Cross-functional collaboration among UX researchers, program managers, and data scientists is essential for responsible AI, requiring a shared understanding of terms like fairness to facilitate effective communication and intervention strategies.


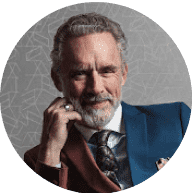
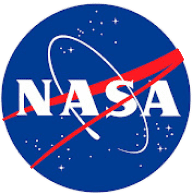
