NVIDIA Gana la BATALLA de la Inteligencia Artificial
Dot CSV・18 minutes read
The battle among major tech companies like Microsoft and Google for AI dominance has driven Nvidia's evolution from graphics hardware to powerful GPUs for neural network training, revolutionizing deep learning with advancements like CUDA and the H100 architecture. Nvidia's push for more powerful GPUs, advancements in AI models, and tools like Chat with RTX and Stable hint towards a future where local execution of advanced AI tasks becomes more accessible and prevalent for both consumers and professionals.
Insights
- Nvidia's strategic shift towards general-purpose GPU use, particularly for AI applications, has revolutionized the field by significantly accelerating neural network training, showcasing the potential of artificial neural networks for various industries.
- The evolution of GPUs, especially Nvidia's H100 architecture, has led to a thousandfold increase in performance, driving a surge in demand for powerful GPUs in AI training and consumer hardware adaptation, indicating a future trend towards more powerful GPUs with advanced technologies for enhanced user experiences and local execution.
Get key ideas from YouTube videos. It’s free
Recent questions
What companies are engaged in the battle for AI dominance?
Microsoft and Google
How did Nvidia revolutionize GPU usage?
Shifted to general-purpose use
What is Jensen Juan's law?
Predicted GPU performance doubling annually
How have GPU training times evolved for AI models?
Drastically reduced over the last five years
What are the future trends in AI computing?
Shift towards open source and capable models
Related videos
60 Minutes
Nvidia CEO Jensen Huang and the $2 trillion company powering today's AI | 60 Minutes
NVIDIA
GTC March 2024 Keynote with NVIDIA CEO Jensen Huang
CNBC
How Nvidia Grew From Gaming To A.I. Giant, Now Powering ChatGPT
Ticker Symbol: YOU
GET IN EARLY! I'm Investing In This HUGE AI Chip Breakthrough
Stanford Institute for Economic Policy Research (SIEPR)
Keynote by NVIDIA CEO Jensen Huang at 2024 SIEPR Economic Summit
Summary
00:00
AI Companies Battle for Powerful GPUs
- In the world of Artificial Intelligence, a fierce battle is ongoing among major companies like Microsoft and Google for both existing and emerging markets.
- Nvidia, originally focused on hardware for computer graphics, shifted towards general-purpose use of GPUs, leading to the development of CUDA for easier programming.
- The use of GPUs for parallel processing significantly accelerated the training of neural networks, leading to the Deep learning revolution.
- GPUs proved crucial in training large neural networks like AlexNet, showcasing the potential of artificial neural networks.
- Jensen Juan's law predicted a doubling of GPU performance annually, surpassing Moore's Law limitations and enabling advancements in parallel computing for AI.
- Over the last five years, GPU training times for models like GPT-4 have drastically reduced, with performance improvements multiplying by 30x.
- The evolution of GPUs, from P1 to H100 architecture, has seen a thousandfold increase in performance, meeting the demand for AI computing.
- Companies are racing to acquire Nvidia's H100 GPUs for AI training, with a significant shift towards more powerful computing centers.
- Consumer hardware, like the RTX 3060, is adapting to the AI wave through enhancements in tensor cores for neural network processing and increased memory capacity.
- Future trends suggest a shift towards more Open source and capable AI models, necessitating powerful GPUs with ample memory for local execution and advanced technologies like DLSS for enhanced user experiences.
16:04
Advancements in AI Tools by Nvidia
- Tools for creating 3D images, videos, and music are advancing rapidly, with local execution becoming more prevalent. Nvidia recently introduced accelerated tools like Stable through their Tensor RT library, enabling video diffusion on Nvidia GPUs seven times faster than on an Apple M2 processor.
- Open-source language models are improving, offering performance comparable to private models like GPT-4. This progress suggests a future where programming with advanced models may be done locally for free, reducing reliance on paid services like OpenAI.
- Nvidia is well-positioned to meet the growing demand for AI-based functionalities in consumer and professional markets. They are developing tools like Chat with RTX, allowing users to interact with Open-source language models locally.
- Nvidia envisions the future of the video game industry with concepts like neural non-playable characters (NNPC), where AI generates real-time character interactions. The implementation of such features may involve running models locally or in the cloud, depending on hardware capabilities and company strategies.


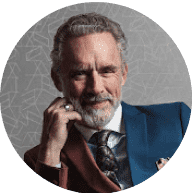
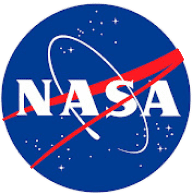
