Data Quality Explained
IBM Technology・1 minute read
Data quality is essential for business success, with accuracy, completeness, consistency, and uniqueness being the key attributes that help maintain a positive reputation. Utilizing machine learning and AI can significantly enhance data quality by automating the assessment process, thereby saving time and improving reliability.
Insights
- Data quality is vital for business success, with four key attributes—accuracy, completeness, consistency, and uniqueness—acting like essential ingredients for a chef; poor data can harm a company's reputation by misrepresenting information, such as failing to account for bot traffic or having inconsistent zip code formats across teams.
- Businesses can significantly improve data quality and minimize manual checks by leveraging machine learning and AI technologies, which automatically evaluate data as it enters the system, enhancing reliability and efficiency while allowing for more informed decision-making.
Get key ideas from YouTube videos. It’s free
Recent questions
What is data quality?
Data quality refers to the overall reliability and accuracy of data used in business processes. It encompasses several key attributes, including accuracy, completeness, consistency, and uniqueness. Accuracy ensures that the data accurately reflects reality, such as accounting for anomalies like bot traffic in lead generation. Completeness means that all necessary information is present, such as names and emails in survey responses. Consistency requires that data is uniform across different sources, avoiding discrepancies like varying formats for zip codes. Uniqueness focuses on eliminating duplicate entries, which can distort the actual figures, such as the number of leads. High data quality is essential for making informed business decisions and maintaining a good reputation.
Why is data accuracy important?
Data accuracy is crucial because it directly affects the reliability of business decisions and outcomes. When data is accurate, it reflects the true state of affairs, allowing companies to make informed choices based on real insights. For example, in lead generation, if a business fails to account for bot traffic, it may overestimate the effectiveness of its marketing efforts, leading to misguided strategies. Accurate data helps in identifying genuine customer behavior and preferences, which is essential for tailoring products and services. Inaccurate data, on the other hand, can lead to poor decision-making, wasted resources, and damage to a company's reputation, highlighting the importance of maintaining high standards of data accuracy.
How can businesses improve data quality?
Businesses can enhance data quality by implementing advanced technologies such as machine learning and artificial intelligence. These technologies can automatically assess and improve the quality of data as it enters the system, significantly reducing the need for manual inspection. By utilizing AI, companies can ensure that data meets the essential qualities of accuracy, completeness, consistency, and uniqueness without extensive human intervention. This not only saves time but also increases the reliability of the data collected, allowing businesses to make better-informed decisions. Additionally, organizations can establish clear data governance policies and regular audits to maintain high data quality standards over time.
What is data completeness?
Data completeness refers to the extent to which all necessary information is present in a dataset. It is a critical aspect of data quality, as incomplete data can lead to misleading conclusions and ineffective business strategies. For instance, in survey responses, if fields such as names and emails are left blank, the analysis may lack essential insights about customer preferences and demographics. Ensuring data completeness involves filling in all required fields and verifying that no critical information is missing. This attribute is vital for accurate reporting and decision-making, as it allows businesses to have a comprehensive view of their data landscape and make informed choices based on complete information.
What does data uniqueness mean?
Data uniqueness refers to the principle of ensuring that each entry in a dataset is distinct and not duplicated. This quality is essential for accurately representing the actual number of entities, such as leads or customers, within a database. When duplicates exist, they can distort metrics and lead to erroneous conclusions, such as overestimating the size of a customer base. Maintaining data uniqueness involves implementing processes to identify and eliminate duplicate records, which can be achieved through data cleansing techniques and validation rules. By focusing on uniqueness, businesses can enhance the integrity of their data, leading to more accurate analyses and better decision-making outcomes.
Related videos
Summary
00:00
Enhancing Business Outcomes Through Data Quality
- Data quality significantly impacts business outcomes, akin to a chef using poor-quality ingredients, which can damage a company's reputation. Four main qualities of data are crucial: accuracy, completeness, consistency, and uniqueness. For instance, accuracy involves ensuring data reflects reality, such as accounting for bot traffic in lead generation; completeness requires all necessary fields to be filled, like names and emails in survey responses; consistency demands uniformity across data sources, such as zip codes formatted differently by various teams; and uniqueness focuses on eliminating duplicates, which can misrepresent the actual number of leads.
- To enhance data quality and reduce manual inspection, businesses can utilize machine learning and AI technologies that automatically assess these data qualities as they enter the system. This approach not only saves time but also improves the reliability of the data collected. For more information on these AI features, links are provided for further exploration, and subscribing to the channel is encouraged for those interested in technology advancements.


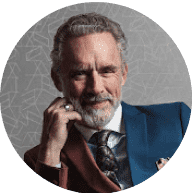
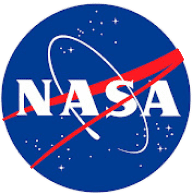
