Build Your Startup With AI
a16z・84 minutes read
The text delves into the negative impact of companies prioritizing profit over safety, using examples like Google and Microsoft in the context of AI. Discussions cover various aspects of AI development, limitations, potential improvements, and ethical considerations, highlighting the need for a balance between human intelligence and AI capabilities.
Insights
- Companies like Google and Microsoft prioritize profits over safety, acquiring resources like GPUs and necessitating government intervention to maintain equitable access, highlighting the negative impact of greed in the pursuit of profit.
- The evolution of AI models and their potential limitations are explored, emphasizing the unique role AI plays in enhancing human intelligence, with discussions ranging from nuanced building techniques to the exploration of AI's ability to discover new concepts like the laws of physics, showcasing the intricate balance between AI advancements and human capabilities.
Get key ideas from YouTube videos. It’s free
Recent questions
How do companies prioritize profit over safety?
Companies prioritize profit over safety by harming society.
What are the implications of AI in company building?
AI in company building intersects with startup development.
How can AI models be improved significantly?
AI models can be improved through supervised learning.
What role does data play in AI training?
Data is a valuable asset for AI training.
How can AI enhance healthcare?
AI can enhance healthcare through detailed diagnostics.
Related videos
Bloomberg Originals
Satya Nadella & Sam Altman: Dawn of the AI Wars | The Circuit with Emily Chang
ColdFusion
AI Deception: How Tech Companies Are Fooling Us
Financial Wise
Eric Schmidt Full Controversial Interview on AI Revolution (Former Google CEO)
Institute for the Future of Work
Using AI in the workplace: ethical risks and policy responses
AltStrip
Mo Gawdat - бывший коммерческий директор Google X. Опасности развития ИИ.
Summary
00:00
"AI, Greed, and Safety: Future Considerations"
- The text discusses the negative impact of companies driven by greed, willing to harm the country and the world for profit, under the guise of safety.
- Google and Microsoft are highlighted as examples of companies pursuing profit at the expense of safety, buying up all available GPUs and needing government intervention to prevent open access.
- The focus of the discussion is on AI in April 2024, particularly its intersection with company building and startup development.
- Questions from Michael, Gwen, and Alist McLay are addressed, covering topics like upcoming AI capabilities, competition for small AI startups against established players, and characteristics of companies benefiting from AI improvements.
- Sam Mman's advice is shared, emphasizing the need for startup founders to consider how much better existing foundation models could become and whether it benefits or threatens their startup.
- Nuances in building foundation models are discussed, highlighting architectural differences, model speed, domain specificity, and the potential for distillation to create smart models at lower costs.
- The debate on whether big AI models will improve significantly is explored, considering factors like sophistication of answers, lack of hallucination, and alignment issues.
- The text delves into the limitations of AI trained on internet data, which tends to reflect average human intelligence, but also contains data from exceptionally smart individuals.
- Crafting prompts for AI can lead to accessing different parts of the data set, potentially unlocking super genius capabilities, as seen in examples like writing secure code or emulating John Carmack's coding style.
- The discussion concludes with considerations on the potential limits of AI intelligence compared to humans, and its ability to discover new concepts like laws of physics, highlighting the unique role AI plays in complementing human intelligence.
14:20
Advancements in AI Training and Capabilities
- Generalized learning occurs within neural networks, evidenced by introspection techniques revealing evolving neural circuitry during training.
- Neural networks can develop general computation functions, as seen in a case where a network trained on chess games created a model of a chessboard and made original moves.
- Meta and others discuss the effectiveness of overtraining, which involves extended training against the same data, leading to improved performance.
- Supervised learning significantly enhances AI capabilities, providing a substantial boost.
- Self-improvement loops are being explored, where AI is trained on answers to problems it has solved, leading to enhanced reasoning capabilities.
- Synthetic data is a topic of debate, with some experts optimistic about its potential benefits.
- AI models may excel at validating code rather than writing it, showcasing a unique dynamic in their capabilities.
- Various improvements in AI are expected, including resolving chip constraints, generating new data, and enhancing systems engineering.
- Microsoft's release of a small language model optimized its training set, achieving competitive results with larger models.
- The application layer of AI faces challenges in transitioning from AI co-pilots to pilots, necessitating human involvement for correctness and process flow understanding.
26:28
"Value-Based Pricing: Enhancing Tech Investments with AI"
- Pricing model based on business value to the customer
- Charge a percentage of the business value, e.g., 10%
- Example of AI company, Crest AI, specializing in debt collection
- Bridging the gap between value and cost
- Importance of charging based on value, not just work
- Difficulty for technology investors with specific solution vendors
- Potential shift in tech investment structure due to AI
- Jevons Paradox: technological progress increases resource efficiency but also demand
- AI's potential to enhance software capabilities and increase demand
- Unlimited potential for new needs and innovations in society
39:55
Maximizing Data Value Through Internal Utilization
- In software, there is always room for improvement, automation, and optimization.
- AI technology has revolutionized security cameras, enabling semantic understanding of environments.
- AI can enhance healthcare by providing detailed diagnostics and health monitoring.
- Data is often considered the most valuable asset for AI training, but its actual value may be overestimated.
- Companies can leverage their data for internal use, like improving investor relations or product optimization.
- The belief that proprietary data is highly valuable may not hold true due to the vast amount of available data on the internet.
- Companies should focus on utilizing their data internally rather than trying to sell it as a valuable asset.
- Actuarial data in insurance companies may have unique value based on individual profiles and lab results.
- Companies like Coinbase may have valuable data specific to their industry that can enhance their operations.
- Enterprises must consider the implications of sharing their data with larger companies and competitors for AI training.
53:35
"Data's Role in AI Revolutionizing Healthcare"
- Access to data on all humans' health, genetics, etc., is crucial in the world of AI.
- Data is likened to the new oil in healthcare, offering insights into sickness causes.
- Locking up data to prevent insurance overcharging may hinder healthcare improvements.
- Subsidizing healthcare differentially for high-risk individuals could be a more effective solution.
- Insurance's concept may be challenged with predictive genomic data, altering risk pooling.
- Health insurance's structure is critiqued for its bureaucracy and inefficiency.
- Societal willingness to pool resources for healthcare may diminish with predictive data.
- Incentivizing healthy behaviors could be more beneficial than simply paying for healthcare.
- The healthcare system requires systemic fixes to be more efficient and fair.
- AI's evolution is compared to the computer industry's growth, emphasizing the shift from few large models to a vast array of smaller ones.
01:05:58
"AI's Impact on Technology Adoption and Innovation"
- The size of a computer needed depends on the user's requirements and tasks.
- AI models will vary in shape, size, capabilities, and training data, creating a diverse ecosystem.
- Historically, computing lock-in stemmed from the complexity of using specific systems.
- AI, being user-friendly, raises questions about potential lock-in to specific models.
- Lessons from the internet era include the boom-bust nature of technology adoption.
- AI's growth may lead to overfunding, resulting in company failures and excess infrastructure.
- The internet transitioned from an open to a closed system, with AI facing a similar choice.
- AI's trajectory could either foster open innovation or be monopolized by existing tech giants.
- The speculative nature of new technologies, like AI, is a common historical trend.
- Speculation in new tech drives innovation and investment, despite risks and failures.


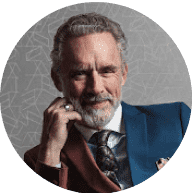
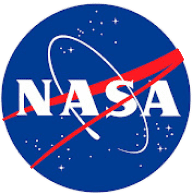
