21. Chaos and Reductionism
Stanford・2 minutes read
Reductionism is vital for understanding complex systems but falls short in explaining individual variations, highlighting the need for a more holistic approach to grasp the full complexity of the system. The study underscores the consistent variability inherent in chaotic systems, challenging traditional reductive scientific expectations and pointing to the intrinsic nature of noise in data across different levels of analysis.
Insights
- Lectures at Stanford University focus on unconventional scientific concepts, challenging due to the unconventional thinking required.
- The standard Western approach to complex systems evolved after Rome's fall, emphasizing reductionism for predictability.
- Chaos theory posits that variability in chaotic systems is intrinsic, leading to unpredictable outcomes despite detailed examination.
Get key ideas from YouTube videos. It’s free
Recent questions
What is reductionism in science?
Breaking down complex systems into simpler parts.
Related videos
TEDx Talks
TEDxMidAtlantic 2011 - Duncan Watts - The Myth of Common Sense
TED
Benoit Mandelbrot: Fractals and the art of roughness
The Royal Institution
Chaos theory and geometry: can they predict our world? – with Tim Palmer
TEDx Talks
Potential: Jordan Peterson at TEDxUofT
André Duqum
Proof That Reality Is An ILLUSION: The Mystery Beyond Space-Time - Donald Hoffman | Know Thyself E63
Summary
00:00
"Complex Science: Evolving Perspectives and Predictive Systems"
- Lectures at Stanford University focus on complex scientific concepts, particularly challenging due to the unconventional thinking required.
- The Chaos book is recommended for its ability to provoke passionate reactions and challenge traditional scientific perspectives.
- A homework assignment involving creating cellular automata is given, emphasizing intense focus and time dedication.
- The standard Western approach to understanding complex systems began to evolve after the fall of Rome and the subsequent rediscovery of knowledge in Toledo's library.
- The emergence of syllogistic thinking and the ability to reconstruct events from overlapping data marked a significant shift in intellectual progress.
- Reductionism, the core concept of modern science, involves breaking down complex systems into simpler parts for understanding.
- Predictability in reductive systems allows for the accurate prediction of complex system outcomes from known starting states.
- Extrapolation, based on the additivity of component parts, enables the prediction of outcomes without detailed calculations.
- Blueprints are essential in reductive systems for quality control and guidance, ensuring the desired outcome is achieved.
- Variability in measurements is acknowledged, with averages representing a range of values around a central point like the normal human body temperature of 98.6 degrees Fahrenheit.
15:34
Reducing Variability Through Reductive Analysis
- Variability is considered noise in a reductive view of science, seen as junk in the system and something to eliminate.
- In this perspective, noise represents instrument error, indicating imperfections in the system used for measurement or observation.
- The solution to reducing variability lies in becoming more reductive, focusing on detailed examination to eliminate noise.
- The closer one looks at a phenomenon with advanced techniques, the less variability is expected to be present.
- Examples include measuring body temperature: using a Zeppelin is more variable than using a thermometer held to the forehead.
- Reductionism is a key concept, aiming to break down complex systems into simpler components to eliminate variability.
- Neurobiologists Hubel and Wiesel demonstrated a reductive system in the visual cortex, where individual neurons responded to specific stimuli.
- Neurons in different layers of the cortex were specialized in recognizing different visual elements, showcasing a hierarchical reductive process.
- The expectation was to find neurons in higher layers that could recognize complex sensory information, like faces or symphonies.
- While the idea of "grandmother neurons" was popular, few actual instances have been found, with sparse coding showing limited existence of such specialized neurons.
29:40
"Neurons, Faces, and Complexity in Biology"
- A neuron was found to respond specifically to a picture of the Sydney Opera House, showcasing its unique characteristics.
- The concept of grandmother neurons, which respond to specific stimuli like Jennifer Aniston, faces a challenge due to the brain's limited number of neurons.
- The complexity of recognizing faces requires a vast number of neurons, surpassing the brain's capacity.
- Neural networks, rather than reductive processing, are now favored for understanding complex information processing in the brain.
- Bifurcating systems, like neuronal dendrites and blood vessels, exhibit scale-free complexity and are crucial in living organisms.
- The intricate branching patterns in neurons and blood vessels pose a challenge for reductive coding due to the vast scale differences.
- The coding for bifurcating systems in living organisms cannot be achieved through a reductive approach due to the limited number of genes.
- Chance plays a significant role in biological systems, affecting outcomes like dominance hierarchies in fish colonies.
- Reductionism fails to predict complex biological functions due to the influence of chance and the inability to account for all variables.
- The limitations of reductive approaches in understanding brain function and genetic regulation highlight the need for alternative methods beyond classical reductionism.
43:53
Understanding Chaotic Systems: Nonlinear, Unpredictable, Complex
- Chaotic systems are non-reductive, with non-linear nonadditivity, unlike fixing a clock by identifying and replacing missing parts.
- Reductive approaches work for fixing clocks but not for understanding complex systems like clouds during a drought.
- Complex biological systems are like clouds, not clocks, requiring a different explanatory system.
- Westernized reductionism is effective for simple systems but inadequate for complex, interesting systems due to insufficient components and the role of chance.
- Chaotic systems are non-linear and nonadditive, with pieces not adding up in a linear manner, leading to unpredictable outcomes.
- Deterministic systems can be periodic or aperiodic, with periodic systems showing predictability through repeated application of rules.
- Aperiodic systems have rules but lack constant relationships between steps, requiring stepwise application of rules for prediction.
- Chaotic systems are deterministic but non-linear, with relationships between steps being different, making prediction challenging without going through calculations.
- Water wheel example illustrates how increasing force can lead to a shift from periodic to chaotic patterns, generating unpredictable, non-repeating outcomes.
- Chaotic systems result from increased force, leading to patterns that never repeat, showcasing infinite variability due to the system's chaotic nature.
57:40
"Chaos and Predictability in Complex Systems"
- To predict events 4,000 years ahead, studying the wheel's behavior over time is necessary.
- Chaos arises in structured systems when force increases, leading to unpredictability and non-repeating patterns.
- Chaotic systems, like water wheels under heat, transition from periodicity to chaos, with no predictability.
- Periodicity of an odd number indicates entry into chaotic terrain, as seen in water wheel convection patterns.
- Chaotic systems lack predictability, requiring continuous observation to understand future patterns.
- Chaosists argue that complex systems operate in chaotic realms, often ignored by science focusing on linear periodic systems.
- Linear systems have attractors that pull the system back to stability after perturbations.
- Chaotic systems exhibit strange attractors, causing constant oscillation without settling into a stable point.
- In chaotic systems, variability is not noise but the system's essence, with no idealized answer.
- The butterfly effect in chaotic systems amplifies tiny differences, leading to significant consequences, as seen in the weather system.
01:11:41
"Chaos Theory: Unpredictable Systems and Fractals"
- In chaotic systems, even a small change, like a butterfly flapping its wings, can lead to significant differences in outcomes.
- The pattern in chaotic systems never repeats, making predictability impossible.
- Chaos theory suggests that variability is intrinsic to the system and will amplify consequences unpredictably.
- The concept of a strange attractor in chaotic systems indicates that there is no definitive answer or predictability.
- Variability in chaotic systems remains constant regardless of the scale or level of detail examined.
- Fractals are complex patterns or systems that exhibit scale-free properties, meaning their complexity remains constant at any level of observation.
- Fractals have fractional dimensions and exhibit infinite complexity, making them unique and not easily categorized.
- Fractals are found in various systems, such as the circulatory system, pulmonary system, dendrites of neurons, and tree branches.
- Fractal systems display consistent variability independent of scale, indicating that noise is an inherent part of the system.
- A study on the effects of testosterone on behavior revealed that variability in data does not decrease with increased reductionism, contrary to reductive scientific expectations.
01:26:11
Fractal Interpretation Reveals Intrinsic Data Variability
- The chaotic fractal interpretation suggests that noise in data is actually intrinsic oscillatory information within the system, not something to eliminate with better tools.
- This interpretation predicts that the variability in data should not decrease with different levels of examination, indicating a consistent level of variability across scales.
- Extensive research in the early 1990s produced a figure showing a consistent coefficient of variation across different levels of analysis, from organismal to subcellular.
- The study encompassed data from various fields, ranging from societies to crystallography, revealing that data variability remains constant due to the fractal nature of the system.
- Analyzing influential papers from a decade prior helped distinguish high-quality research from lower-quality work, demonstrating that even top-tier papers exhibited consistent variability.
- Despite the groundbreaking findings, the research faced challenges in publication, eventually being accepted by a journal focused on philosophical discussions in medicine and biology.
- The study's impact was minimal in terms of citations, except for a mathematician in Moscow who greatly appreciated the work.
- The text highlights the limitations of classical reductionism in understanding complex systems, emphasizing the need for a more holistic approach.
- While reductionism is useful for general predictions and practical applications, it falls short in explaining individual variations within complex systems, necessitating a broader perspective for comprehensive understanding.


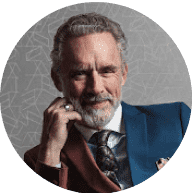
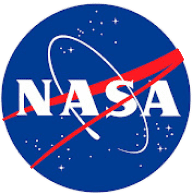
