Math 120: Statistics --- Chapter 7: The Central Limit Theorem
VVC Schellhous・2 minutes read
Chapter 7 explains the Central Limit Theorem and its application to sample means, emphasizing the normal distribution and sampling distributions. The Central Limit Theorem asserts that sample means are normally distributed for large sample sizes, using the population mean and standard deviation to determine the sampling distribution characteristics.
Insights
- The Central Limit Theorem asserts that sample means become normally distributed for large sample sizes, with the mean being the same as the population mean and the standard deviation being the population standard deviation divided by the square root of the sample size.
- Sampling distributions, particularly for sample means, play a crucial role in statistics, showcasing how multiple samples of the same size yield different means, emphasizing the importance of understanding the variability inherent in sample statistics.
Get key ideas from YouTube videos. It’s free
Recent questions
What is the Central Limit Theorem?
A statistical concept about sample means and distributions.
How are sample means determined?
By summarizing samples from populations using statistics.
What is the relationship between sample size and sampling distribution?
Sample size affects the shape and characteristics of the sampling distribution.
How does the Central Limit Theorem apply to different populations?
The Central Limit Theorem applies universally with certain conditions.
How can the Central Limit Theorem be used in practice?
By applying it to sample means and sums of data values.
Related videos
Vedantu Telugu
Statistics | One Shot Revision | Class 10 | Haripriya Mam | Vedantu Telugu
JEE Nexus by Unacademy
Binomial Theorem Class 11 | One Shot | JEE Main & Advanced | Arvind Kalia Sir
Tech Classes
Complete STATISTICS for Data Science | Data Analysis | Full Crash Course
PW Little Champs 6th, 7th & 8th
Playing with numbers || Full Chapter in 1 Video || Class 6th Maths || Champs Batch
Rajat Arora
Collection of Data | Chapter 2 | Introduction | Class 11 | ONE SHOT
Summary
00:00
Central Limit Theorem and Sampling Distributions
- Chapter 7 covers the Central Limit Theorem, building on Chapter 6 about the Normal Distribution.
- The lesson focuses on using the Normal Distribution to analyze sample means, not new probabilities.
- Sampling distributions are key, especially for sample means, derived from various statistics.
- Sample statistics, like the mean denoted as x bar, summarize samples from populations.
- Multiple samples of the same size from a population yield different sample means due to chance.
- Sample means are random variables determined by the sampling process.
- The sampling distribution is a probability distribution of sample means from various samples.
- The mean of sample means equals the population mean, while the standard deviation is related.
- The standard deviation of sample means is the population standard deviation divided by the square root of the sample size.
- An example with students watching TV illustrates how to find the mean and standard deviation of a sampling distribution.
18:41
Normal Distribution in Sampling Distributions
- The probability histogram for the sampling distribution shows a bell-shaped curve, indicating a normal distribution.
- The sampling distribution of sample means tends towards a normal distribution as sample size increases.
- A skewed population's sampling distribution becomes more normal with larger sample sizes.
- For a sample size of 30, the sampling distribution closely resembles a normal curve.
- The Central Limit Theorem states that sample means are normally distributed for large sample sizes.
- The mean of the sampling distribution is the same as the population mean, while the standard deviation is the population standard deviation divided by the square root of the sample size.
- The Central Limit Theorem applies to all populations, regardless of their distribution, with a sample size greater than 30.
- If the population is normal, the Central Limit Theorem applies regardless of sample size.
- The Central Limit Theorem can be used for sample means if the sample size is greater than 30 or if the population is normal.
- The Central Limit Theorem also applies to sums of data values, with the mean being the population mean multiplied by the sample size and the standard deviation being the population standard deviation multiplied by the square root of the sample size.
36:54
College Student Age Distribution and Probability
- Mean age of college students in 2011 was 25 years old, with a population standard deviation of 9.5 years.
- A sample of 125 students was drawn, allowing the use of the normal curve due to the sample size being greater than 30.
- The mean of the sampling distribution is 25, with a standard deviation of 9.5 divided by the square root of 125, resulting in 0.76.
- Probability of the sample mean being over 26 years was found to be 0.1197 by calculating the area to the right of 26 in the normal distribution.
- For Hereford cattle, the mean weight of a one-year-old bull was 1135 pounds, with a standard deviation of 97 pounds.


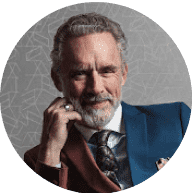
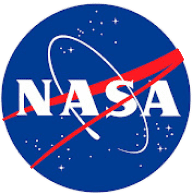
