Lecture 5: Conditioning Continued, Law of Total Probability | Statistics 110
Harvard University・2 minutes read
The discussion focused on conditional probability, problem-solving strategies, and the law of total probability, emphasizing the importance of Bayesian thinking in updating probabilities. Examples involving card hands and medical tests illustrated the application of Bayes' rule and the distinction between conditional and unconditional independence in probability calculations.
Insights
- Breaking down problems into simpler pieces is a key strategy for effective problem-solving, emphasizing the importance of conditional thinking and updating probabilities based on evidence.
- Distinguishing between conditional and unconditional independence is crucial in probability calculations, as demonstrated through examples like game outcomes and fire alarms, highlighting the complexity of real-life scenarios and the need for precision in understanding these concepts.
Get key ideas from YouTube videos. It’s free
Recent questions
What is the importance of conditional probability?
Conditional probability is crucial for updating probabilities based on evidence and breaking problems into simpler pieces. It allows for a more accurate assessment of probabilities by considering specific conditions or information that may affect the outcome. Understanding conditional probability is essential in various fields, including statistics, machine learning, and decision-making processes, as it provides a framework for making informed decisions based on available evidence.
How can problem-solving strategies be enhanced?
Problem-solving strategies can be enhanced by trying simple and extreme cases, breaking problems into simpler pieces, and choosing a useful partition for problem-solving. By breaking down complex problems into more manageable components, individuals can approach problem-solving in a systematic and effective manner. Practice and experience play a significant role in developing problem-solving skills, as they allow individuals to identify patterns, apply different strategies, and improve their overall problem-solving abilities.
What is the law of total probability?
The law of total probability involves partitioning a set into disjoint pieces to calculate the overall probability of an event. By considering all possible outcomes within a given set and their respective probabilities, the law of total probability provides a comprehensive approach to determining the likelihood of an event occurring. Choosing a useful partition is essential in applying this law effectively, as it helps in breaking down complex problems into simpler components and obtaining accurate probability estimates.
Why is Bayes' rule important in probability calculations?
Bayes' rule is crucial in updating probabilities based on evidence and maintaining coherency in probability calculations. It allows individuals to revise their initial beliefs or probabilities based on new information or evidence, leading to more accurate and informed decisions. By incorporating Bayes' rule into probability calculations, individuals can adjust their probabilities in a logical and consistent manner, ensuring that their conclusions are based on the most up-to-date information available.
What is the difference between independence and conditional independence in probability?
Understanding the distinction between independence and conditional independence is crucial in probability calculations. Independence refers to events that are unrelated and do not influence each other's outcomes, while conditional independence occurs when events are independent given specific conditions or information. Recognizing the difference between these concepts is essential in accurately assessing probabilities and making informed decisions based on the relationships between different events. By distinguishing between independence and conditional independence, individuals can navigate complex scenarios and analyze the interplay between various factors affecting the likelihood of specific outcomes.
Related videos
Harvard University
Lecture 4: Conditional Probability | Statistics 110
Mathe by Daniel Jung
1. & 2. Pfadregel in der Wahrscheinlichkeitsrechnung | Mathe by Daniel Jung
Harvard University
Lecture 7: Gambler's Ruin and Random Variables | Statistics 110
Wil MacDonald
Practice Test Probability Grade 8
3Blue1Brown
Bayes theorem, the geometry of changing beliefs
Summary
00:00
"Conditional Probability and Problem-Solving Strategies"
- Bayes Rule was discussed in the previous session, along with the importance of conditional probability.
- The focus of the current discussion is on probability and thinking, emphasizing the need for conditional thinking.
- Problem-solving strategies were highlighted, including trying simple and extreme cases and breaking problems into simpler pieces.
- A general strategy for solving problems involves breaking them into simpler pieces, which is crucial for effective problem-solving.
- The concept of partitioning a set into disjoint pieces was explained, leading to the law of total probability.
- The importance of choosing a useful partition for problem-solving was emphasized, requiring practice and experience.
- Conditional probability was discussed as essential for updating probabilities based on evidence and breaking problems into simpler pieces.
- Two examples of conditional probability involving random card hands were presented for practice.
- The probability of both cards being aces, given that one card is an ace, was calculated using the definition of conditional probability.
- The probability of both cards being aces, given that one card is the ace of spades, was discussed, highlighting different approaches to solving the problem.
15:17
Probability and Bayes' Rule in Testing
- Probability of drawing the jack of diamonds or ace of clubs is 3/51 due to symmetry.
- Simplifying 3/51 gives 1/17, which is twice as likely as 1/34.
- The probability remains 1/17 regardless of the ace's suit.
- Specifying the Ace of Spades doubles the probability to 1/33.
- Conditional probability complexities arise in distinguishing between at least one ace and a specific ace.
- In a medical context, testing for a disease with a 1% affliction rate among similar patients.
- A test advertised as 95% accurate implies a 0.95 probability of correctly detecting the disease.
- Applying Bayes' rule to find the probability of having the disease given a positive test result.
- The law of total probability aids in calculating the overall probability of a positive test result.
- Despite a 95% accurate test, there's only a 16% chance of having the disease, highlighting the importance of second opinions and the impact of disease rarity on intuition.
29:39
"Bayes' Rule: Crucial for Probability Calculations"
- 50 people tested positive without having the disease, while 10 tested positive and did have the disease.
- The importance of considering symptoms and evidence when calculating probabilities is highlighted.
- Bayes' rule is crucial in updating probabilities based on evidence.
- Bayes' rule maintains coherency regardless of the order or timing of evidence received.
- Common mistakes in conditional probability include confusing P(A|B) with P(B|A, known as the prosecutor's fallacy.
- The Sally Clark case exemplifies the dangers of misinterpreting probabilities in legal contexts.
- The prior and posterior probabilities are distinct and must not be confused in calculations.
- Conditional independence, given an event C, is defined as events A and B being conditionally independent.
- Conditional independence does not necessarily imply unconditional independence, as demonstrated in the chess player example.
- Understanding the distinction between independence and conditional independence is crucial in probability calculations.
45:13
Conditional vs. Unconditional Independence in Outcomes
- Game outcomes are conditionally independent, not independent, based on the strength of the opponent, leading to a distinction between conditional and unconditional independence.
- The concept of unconditional independence does not imply conditional independence, as seen in the example of a fire alarm being triggered by either a fire or popcorn, where the events are initially independent but become dependent when the alarm goes off.
- Understanding the difference between unconditional and conditional independence is crucial in scenarios where multiple causes can lead to a single outcome, highlighting the complexity of real-life phenomena.


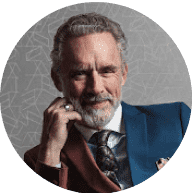
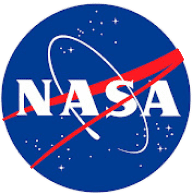
