Lecture 21: Some Examples of Neural Networks
IIT Kharagpur July 2018・21 minutes read
Artificial neural networks are structured with layers of neurons, including input, hidden, and output layers, using different transfer functions and connecting weights denoted by matrices. Training neural networks involves updating weights and transfer function coefficients through iterations, with the steepest descent method used to minimize prediction error through adjustments based on the error gradient and learning rate.
Insights
- Artificial neural networks mimic the structure of biological neurons with layers for input, hidden, and output, utilizing specific transfer functions and connecting weights denoted by matrices V and W.
- Training neural networks involves adjusting connecting weights and transfer function coefficients iteratively to optimize performance, with error calculated between target and actual outputs, minimized using optimization algorithms like the steepest descent method.
Get key ideas from YouTube videos. It’s free
Recent questions
How are artificial neural networks structured?
Artificial neural networks consist of layers of neurons, including input, hidden, and output layers.
What functions are used in neural network layers?
Different transfer functions like linear, log sigmoid, and tan sigmoid functions are used in neural network layers.
How are inputs processed in neural networks?
Inputs are normalized using formulas to scale them to a range of 0 to 1 or -1 to 1 before being multiplied with connecting weights and passed through transfer functions.
What is involved in training neural networks?
Training neural networks includes updating connecting weights and transfer function coefficients through iterations to improve performance.
How is prediction error minimized in neural networks?
Prediction error is minimized using optimization algorithms like the steepest descent method, which updates weights and coefficients based on the error gradient and a learning rate.
Related videos
freeCodeCamp.org
How Deep Neural Networks Work - Full Course for Beginners
Dot CSV
BitNets: La ERA de las REDES NEURONALES de 1 BIT!
DeepBean
Optimization for Deep Learning (Momentum, RMSprop, AdaGrad, Adam)
AI Search
You Don't Understand AI Until You Watch THIS
Quanta Magazine
2021's Biggest Breakthroughs in Math and Computer Science
Summary
00:00
Neural Networks: Structure, Functions, and Training
- Artificial neural networks are designed based on the principles of biological neurons.
- The structure involves layers of neurons, including input, hidden, and output layers.
- Multi-layer feed-forward neural networks consist of input, hidden, and output layers with specific numbers of neurons.
- Different transfer functions are used for each layer, such as linear, log sigmoid, and tan sigmoid functions.
- Connecting weights between layers are denoted by matrices V and W, with values ranging from 0 to 1 or -1 to 1.
- Inputs to neural networks must be normalized to ensure proper functioning.
- Normalization formulas are used to scale inputs to a range of 0 to 1 or -1 to 1.
- Forward calculations involve multiplying inputs with connecting weights and passing them through transfer functions.
- Training neural networks involves updating connecting weights and transfer function coefficients through iterations.
- The performance of neural networks depends on connecting weights, transfer function coefficients, and network architecture.
18:31
Neural Network Output Calculation and Optimization
- The formula for determining the output of a hidden neuron involves a transfer function and coefficients.
- The input of the output layer is calculated using weights and the output of hidden neurons.
- The output of the output layer is determined by passing the input through a transfer function.
- The error between the target output and calculated output is squared and halved for differentiation purposes.
- The total error for all output layer neurons is calculated by summing the individual errors.
- The total error across multiple training scenarios is determined by summing the errors for each scenario.
- To minimize prediction error, an optimization algorithm like the steepest descent method is used.
- The steepest descent method involves updating weights and coefficients based on the error gradient and a learning rate.


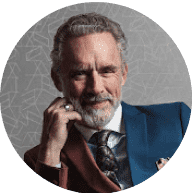
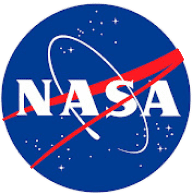
