Detect Your Blood Type with Just a Fingerprint! Using Machine Learning | IEEE 2024 Project
Ieee Xpert・2 minutes read
The project presents a non-invasive method for predicting blood groups using fingerprints, harnessing machine learning techniques that achieve a 98% accuracy compared to the traditional 85%. By utilizing a web application built on the Flask framework, users can easily upload fingerprint images for quick blood group predictions, significantly improving efficiency and safety over conventional testing methods.
Insights
- The project introduces a groundbreaking method for predicting blood groups using fingerprints, offering a non-invasive alternative to traditional blood testing that typically requires blood samples and chemical reactions, thus enhancing safety and efficiency while achieving an impressive accuracy rate of 98% compared to the existing 85%.
- By employing machine learning techniques, specifically a Convolutional Neural Network (CNN), the system analyzes unique fingerprint features to classify blood groups, streamlining the process into an accessible web application where users can upload their fingerprints for immediate results, thereby simplifying the testing experience and reducing the risk of contamination associated with conventional methods.
Get key ideas from YouTube videos. It’s free
Recent questions
What is a blood group?
A blood group is a classification of blood based on the presence or absence of specific antigens on the surface of red blood cells. The main blood groups are A, B, AB, and O, which can be further classified into positive or negative based on the presence of the Rh factor. Understanding blood groups is crucial for blood transfusions, organ transplants, and pregnancy, as incompatible blood types can lead to serious health complications. Each blood group has unique characteristics and compatibility requirements, making it essential for medical professionals to accurately determine a patient's blood type before any medical procedures.
How do I improve my fingerprint security?
To enhance fingerprint security, consider using multi-factor authentication, which combines fingerprint recognition with another form of identification, such as a password or a security token. Regularly update your devices and software to protect against vulnerabilities, and ensure that your fingerprint data is stored securely, preferably in an encrypted format. Additionally, avoid using the same fingerprint for multiple devices or accounts to minimize risks. It's also advisable to keep your fingerprint scanner clean and functional, as dirt or damage can affect its accuracy and reliability. By implementing these measures, you can significantly bolster the security of your fingerprint-based systems.
What is machine learning?
Machine learning is a subset of artificial intelligence that focuses on the development of algorithms and statistical models that enable computers to perform specific tasks without explicit programming. It involves training a model on a dataset, allowing it to learn patterns and make predictions or decisions based on new data. Machine learning is widely used in various applications, including image recognition, natural language processing, and predictive analytics. The technology relies on large amounts of data and computational power to improve its accuracy over time, making it a powerful tool for solving complex problems across different industries.
What are the benefits of non-invasive testing?
Non-invasive testing offers several advantages, including reduced risk of complications, increased patient comfort, and quicker results. Unlike traditional methods that require blood samples or other invasive procedures, non-invasive tests can often be performed with minimal discomfort, making them more appealing to patients. Additionally, these tests can lead to faster diagnosis and treatment, as they eliminate the need for lengthy sample collection and processing. Non-invasive methods also reduce the risk of infection and contamination, contributing to overall safety in medical practices. As technology advances, the development of non-invasive testing continues to improve healthcare accessibility and efficiency.
How does a Convolutional Neural Network work?
A Convolutional Neural Network (CNN) is a type of deep learning model specifically designed for processing structured grid data, such as images. It works by applying convolutional layers that filter input data through learned patterns, allowing the network to detect features like edges, textures, and shapes. These features are then pooled to reduce dimensionality and retain the most important information. The CNN architecture typically includes multiple layers, including convolutional, pooling, and fully connected layers, which work together to classify images or extract relevant features. This approach is particularly effective in tasks like image recognition and classification, achieving high accuracy rates in various applications.
Related videos
Unacademy NEET
Molecular Basis of Inheritance Part 3 | NEET 2024 | Seep Pahuja
RegisteredNurseRN
Blood Types Explained | Blood Groups (ABO) and Rh Factor Nursing Transfusions Compatibility
Two Minute Papers
DeepMind AlphaFold: A Gift To Humanity! 🧬
Sarah Williams
FRSC 202 Serology
Trace Smart Technologies
Breast Cancer Detection using Convolutional Neural Networks (CNN)
Summary
00:00
Fingerprint Analysis for Blood Group Prediction
- The project focuses on using fingerprints to predict blood groups, aiming to provide a non-invasive alternative to traditional blood group testing methods, which typically require blood samples and chemical reactions.
- Blood groups are categorized into six main types: A positive, A negative, B positive, B negative, O positive, and O negative, with some rare blood groups also existing.
- Traditional blood group testing involves collecting blood samples and mixing them with chemicals, which can be time-consuming (taking about 5 to 10 minutes) and requires specialized equipment and materials, posing risks of contamination and requiring significant resources.
- The proposed system collects various fingerprints corresponding to different blood groups to create a dataset, which is then analyzed using machine learning and deep learning techniques to identify unique fingerprint features associated with each blood group.
- The project utilizes a Convolutional Neural Network (CNN) for feature extraction and classification, which is designed to handle image data effectively and is expected to yield higher accuracy compared to traditional methods, achieving an accuracy of 98% in the proposed system versus 85% in existing systems.
- The methodology includes four main steps: dataset collection, preprocessing (normalization and resizing of images), model design (using CNN), and model evaluation, ensuring a comprehensive approach to blood group prediction.
- To implement the project, a minimum of an Intel i3 processor with 4GB of RAM is required, along with a compatible operating system (Windows or Mac), and the coding languages used include Python, HTML, CSS, and JavaScript.
- The web application is built using the Flask framework, allowing users to upload fingerprint images and receive blood group predictions without needing backend processing, enhancing user accessibility.
- Users can create accounts on the web application, where they can sign up and log in to upload their fingerprint images for blood group prediction, with the system storing user data in a database.
- The project demonstrates a significant improvement in efficiency and safety over traditional methods, eliminating the need for blood samples and chemicals, and providing rapid results through a user-friendly interface.
17:53
Project Delivers High Accuracy End-to-End Solution
- The project achieves a 98% accuracy rate, significantly higher than the existing 85% accuracy.
- The training loss is approximately 0.15% for both training and testing phases.
- The project is described as an end-to-end solution.
- For acquisition of the project, contact itexpert.com for the best pricing.


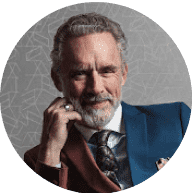
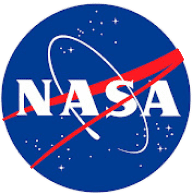
