Dario Amodei (Anthropic CEO) - $10 Billion Models, OpenAI, Scaling, & Alignment
Dwarkesh Patel・2 minutes read
Scaling in AI is an empirical fact without a clear explanation, with challenges in predicting specific abilities in AI models. Various factors like data limitations and loss functions can impact scaling, with a focus on improving model efficiency and addressing safety concerns in AI development.
Insights
- Physics concepts like power law of correlations may offer insight into the empirical fact of scaling in AI, despite the unexplained smooth scaling with parameters and data amounts.
- The gap between specific task proficiency and general intelligence in AI models poses a challenge, prompting questions about scaling models versus adding new objectives like RL for efficiency.
- Challenges in integrating AI into existing systems, optimizing deployment, and balancing training and testing sets for alignment methods are crucial for overcoming biases and ensuring effectiveness.
Get key ideas from YouTube videos. It’s free
Recent questions
What is the significance of scaling in AI?
Scaling in AI refers to the process of increasing the size and complexity of AI models to improve their performance and capabilities. It is an empirical fact that has been observed in the field, where larger models tend to exhibit better results. Despite this phenomenon, there is no clear explanation for why scaling works so effectively in AI. The concept of scaling is crucial for advancing AI technology and achieving breakthroughs in various tasks and domains.
How does mechanistic interpretability contribute to AI models?
Mechanistic interpretability plays a vital role in understanding how AI models function and why they exhibit certain behaviors. By delving into the internal processes of these models, researchers can gain insights into the sudden emergence of new abilities, such as addition in language models. This interpretability is essential for ensuring that AI models align with desired values and goals, as well as for identifying potential risks or limitations in their performance. Overall, mechanistic interpretability is a key aspect of developing safe and effective AI systems.
What are the potential reasons for scaling plateaus in AI?
Scaling plateaus in AI refer to points where increasing the size or complexity of models no longer leads to significant improvements in performance. These plateaus may occur due to various factors, such as limitations in available data, architectural constraints, or challenges in optimizing loss functions. Understanding and addressing these plateaus is crucial for continuing the advancement of AI technology and overcoming barriers to achieving higher levels of performance and efficiency.
How do loss functions like RL impact training models?
Loss functions, such as reinforcement learning (RL), play a critical role in training AI models by guiding them towards desired outcomes and behaviors. In the context of scaling AI, these loss functions can be used to replace traditional methods like next token prediction to improve the efficiency and effectiveness of training processes. By optimizing loss functions, researchers can enhance the performance of AI models across various tasks and domains, ultimately leading to more advanced and capable systems.
What are the challenges in integrating AI into existing systems?
Integrating AI into existing systems poses various challenges, including inefficiencies in initial deployment, the need for optimization over time, and obstacles in adapting workflows to accommodate AI technologies. Despite the rapid advancements in AI capabilities, the process of integrating these technologies into real-world applications can be complex and time-consuming. Overcoming these challenges is essential for unlocking the full potential of AI and maximizing its impact on industries and society as a whole.
Related videos
World Science Festival
AI: Grappling with a New Kind of Intelligence
Center for Humane Technology
The A.I. Dilemma - March 9, 2023
World Science Festival
AI and Quantum Computing: Glimpsing the Near Future
Curiosity Stream
AI Tipping Point | Full Documentary | Curiosity Stream
Dhruv Rathee
The Truth about Artificial Intelligence and ChatGPT | Dhruv Rathee
Summary
00:00
"Scaling in AI: Challenges and Insights"
- Dario Amodei, CEO of Anthropic, discusses the phenomenon of scaling in AI
- Scaling in AI is an empirical fact without a clear explanation
- Physics concepts like power law of correlations may offer some insight into scaling
- The smooth scaling with parameters and data amount remains unexplained
- Predicting specific abilities in AI models is challenging
- Mechanistic interpretability attempts to explain sudden emergence of abilities like addition in models
- Alignment and values may not naturally emerge with scaling in AI
- Potential reasons for scaling plateaus include data limitations or architectural issues
- Various loss functions like RL could replace next token prediction for training models
- Data availability is not expected to be a constraint for scaling in AI
14:29
Observing improvement in AI language models.
- Between 2014 and 2017, the speaker observed consistent improvement in various areas, not just speech.
- The speaker noticed patterns of improvement in Dota and robotics, despite data limitations.
- People often focus on immediate problem-solving rather than scaling up solutions.
- The speaker's interest in language models stemmed from their ability to predict the next word.
- The speaker was convinced of the potential of language models after observing GPT-1's capabilities.
- The speaker believed scaling up language models could lead to solving various tasks efficiently.
- Despite impressive model performance, there is a gap between specific task proficiency and general intelligence.
- The speaker questioned whether scaling up models or adding new objectives like RL was more efficient.
- Intelligence is not a linear spectrum but comprises various domain expertise and skills.
- The speaker predicted that AI models would continue to improve across different tasks, potentially reaching human-level intelligence in some areas soon.
28:39
Challenges and Potential of AI Development
- Safety thresholds and government restrictions could prevent rapid progress in AI development.
- Logistical and economic scalability for AI is close, but existential dangers may still exist.
- AI models may not yet be at the threshold to dominate AI research or significantly impact the economy.
- Technical capabilities of AI are advancing rapidly, potentially nearing human-level understanding.
- AI may pass a Turing Test but struggle to contribute significantly to the economy due to skill thresholds and frictions in implementation.
- Challenges in integrating AI into existing systems and workflows may hinder its full potential.
- Deployment of AI systems is often inefficient initially, requiring time to optimize and overcome obstacles.
- AI models are improving rapidly due to increased funding and technological advancements.
- Anthropic's investment in AI research raises questions about costs, benefits, and potential impacts on the industry.
- AI models possess vast knowledge but may struggle to make groundbreaking discoveries due to skill levels and complexities in certain fields like biology.
42:12
"Security and Alignment in AI Training"
- Asilomar conference in the 1970s focused on recombinant DNA, highlighting the emerging possibilities and the importance of careful consideration.
- Organizations are judged not just on their posts but also on their credibility and hype production.
- Uncertainty surrounds the danger of certain messages, emphasizing the need for caution.
- Anthropic emphasizes cybersecurity and innovative architectural measures for efficient training.
- Security measures include limiting knowledge of compute multipliers to essential personnel to prevent leaks.
- Anthropic aims for high security standards, surpassing those of similar-sized companies.
- Mechanistic interpretability is crucial for understanding alignment in AI models.
- Current methods for alignment involve fine-tuning models without full knowledge of internal processes.
- The challenge lies in verifying alignment methods for different scenarios beyond current tasks.
- Balancing training and testing sets for alignment methods is essential to avoid bias and ensure effectiveness.
55:36
"Studying brain circuits for AI safety"
- The text discusses the importance of studying brain circuits at a detailed level to build knowledge.
- Chris Olah is mentioned as a key figure in controlling the interpretability agenda.
- Anthropic's focus is on scaling models and ensuring safety on top of those models.
- Talent density is emphasized over talent mass in Anthropic's approach.
- The text highlights the necessity of frontier models for safety research and the cost associated with them.
- Safety research is tied to scale, with the need for advanced models to ensure effectiveness.
- Misuse and misalignment of AI models are discussed as potential risks, with the need to address both issues.
- The text emphasizes the importance of solving misuse and misalignment issues for a positive future.
- Government involvement is suggested in managing superhuman AI models to ensure responsible control.
- The Long Term Benefit Trust is described as a body that makes decisions for Anthropic, with a focus on AI alignment, national security, and philanthropy.
01:09:04
"Future AI Governance and Security Challenges"
- The need to consider governmental bodies and structures to address new problems is highlighted.
- Discussion shifts to envisioning a future where all major global issues have been resolved by 2030.
- Concerns arise about the potential implications of superhuman AI and the necessity for centralized control until safety issues are resolved.
- Emphasis is placed on the importance of solving safety problems and externalities before determining societal norms.
- The potential role of China in AI development is discussed, with a focus on their efforts to catch up with the US.
- Cybersecurity measures are crucial in preventing state-level actors from accessing sensitive AI information.
- Contemplation on the physical location and security measures required for building AGI is raised.
- The difficulty in predicting the timeline for achieving alignment in AI models is acknowledged.
- The challenges of controlling powerful AI models and the potential risks they pose are highlighted.
- The importance of developing methods to train models effectively and increase interpretability to prevent unintended consequences is emphasized.
01:22:20
"AI Safety: Challenges and Solutions Explored"
- The abstract stories may inspire or provide insight into persistent problems.
- There are concerns about overconfidence in safety work assumptions.
- The possibility of unforeseen disasters is acknowledged.
- Mechanistic interpretability is considered crucial for understanding AI models.
- Specific methods like RLHF and Constitution AI are being researched.
- The need for a basic constitution for AI models is highlighted.
- Concerns about lab leaks and model capabilities are discussed.
- The importance of cybersecurity in AI development is emphasized.
- The necessity for secure data centers and GPUs for future models is mentioned.
- Challenges in procuring components for next-generation models are being addressed.
01:36:24
Model Efficiency and Human Brain Discrepancy Explained
- Estimates suggest GPT-4 performs at around 10^25 Flops, while the human brain's capacity from birth to age 20 is approximately 10^14 Flops.
- Models are significantly smaller than the human brain, requiring three to four orders of magnitude more data for training.
- Human exposure to words until age 18 is in the hundreds of millions, contrasting with models needing hundreds of billions to trillions.
- The efficiency discrepancy between models and the human brain remains a mystery, with models needing more data despite being smaller.
- Factors affecting model efficiency include the number of parameters, model scale, compute power, data quantity and quality, and loss function.
- Architectural symmetries play a crucial role in model efficiency, with limitations in LSTM's ability to attend to the entire context.
- Conditioning and setting up models to allow uninhibited compute flow are essential for optimal performance.
- The potential for future algorithmic progress lies in removing hindrances from older architectures rather than increasing compute power.
- The integration of models into productive supply chains may involve extended tasks and interactions between models and humans.
- The rapid progress in AI poses challenges in predicting economic outcomes and the timing of large revenues from AI products.
01:49:45
Physicists join AI, raise consciousness and safety
- Physicists are able to quickly learn machine learning (ML) and contribute effectively, with many physicists hired by Anthropic.
- Anthropic's recruitment of physicists into AI may divert individuals who would have pursued physics or finance, potentially impacting the ecosystem.
- The development of ML models raises questions about consciousness and AI safety, with concerns about future implications.
- The understanding of intelligence has evolved with the realization that intelligence can be created through specific conditions and signals.
- The CEO of Anthropic prefers a low-profile approach to avoid external influences on his intellectual pursuits and decision-making.
- The CEO criticizes the trend of personalizing companies and CEOs, advocating for focus on the institution's merits rather than individual personalities.


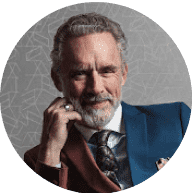
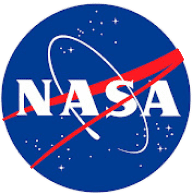
